Introduction
This is an imitation of Anthropic’s
Scaling monosemanticity
work, except using a far smaller language model, roneneldan/TinyStories-33M
.
Code here
Demo
I trained a sparse autoencoder with 10000 features, and GPT-4o says feature 87 relates to
the concepts of upward motion or reaching a peak state such as ‘up’, ‘fly’, ‘high’, and similar directions or motions
Let’s ask the TinyStories model to complete a story starting with “There once was a cat”. I’ll nudge each activation (specifically those just after layer 2) in the direction of feature 87.
And indeed, the cat flies:
There once was a cat named Mittens. Mittens was very fast and loved to play with his friends. One day, Mittens saw a bird fly in the sky like an orange taxi.
Mittens said to his friend, “I wish I could fly like a bird.” The bird replied, “That’s easy, just go slow and never be foolish sometimes.”
Mittens practiced every day, getting better and better. One day, they passed each other and Timmy said, “Wow, you’re so good at flying!” Mittens’ friends laughed and said, “We are like angels!”
From that day on, Mittens was never lazy again and always remembered to practice and race with other kids. He felt happy and free while flying high in the sky.
<|endoftext|>
Training an autoencoder
Let’s forget about the sparse part for now. What we want is an autoencoder: a neural network that takes some input and tries to outputs the same thing.
Q: That sounds like it’s trying to do nothing.
A: Yes, but the autoencoder does it in a complicated way. More on this below
What’s the input?
Anthropic trained on the activation after a middle layer of Claude:
we focused on applying SAEs to residual stream activations halfway through the model (i.e. at the “middle layer”).
They have various justifications for this. My intuition is that the middle layer is maybe where the model is thinking the most about concepts, because at the front and back it has to think more about processing input and preparing output, respectively. (See also this paper: “the middle layers [focus] on more abstract patterns”.)
TinyStories-33M has
4 transformer layers. So we
take the activation after the 2nd
layer, while feeding it short stories
from the roneneldan/TinyStories
dataset.
This is a vector in \( \mathbb{R}^{768} \),
i.e. a list of activation strengths, one for each of 768 neurons.
What’s the autoencoder?
If the autoencoder did nothing, that would be
def autoencoder(llm_activations):
return llm_activations
It’s a little more complicated than that:
def autoencoder(llm_activations):
features = relu(encoder_linear(llm_activations))
return decoder_linear(features)
In this function:
- ReLU maps negative elements to 0
encoder_linear
is an affine transformation \( x \mapsto Ax+b \), where \(A\) is the weight matrix and \(b\) is the bias vectordecoder_linear
is another affine transformation (with different weight \(C\) and bias \(d\))
Q: Why does this have the fancy name autoencoder? It’s just a standard MLP.
A: Because there are some more constraints:
encoder_linear
is \( \mathbb{R}^{768} \to \mathbb{R}^F \), where F is the hidden dimension (F for number of Features) of the autoencoder. That is,encoder_linear
takes a vector with 768 elements, and spits out a vector with F elementsdecoder_linear
is \( \mathbb{R}^{F} \to \mathbb{R}^{768} \). So the net effect is thatautoencoder
returns a vector with the same dimension as the input vector
We’d like activations
and autoencoder(activations)
to be as close together
as possible (“auto” means “self”, not “car”).
This won’t happen by default, since encoder_linear
and decoder_linear
are random transformations.
But we can quantify how far apart they are using an L2 norm:
reconstruction_loss = ((activations - autoencoder(activations)) ** 2).sum()
And then we can use gradient descent to adjust the weights and biases of the autoencoder to minimize the reconstruction loss.
Training
Let’s train autoencoders with different numbers of features:
- \(F=100\) (green)
- \(F=767\) (blue)
- \(F=768\) (red)
- \(F=1000\) (orange)
We’ll save checkpoints every 5000 steps because we’ll do some inference later.
Pytorch makes it easy to save models
using torch.save(model, path)
.
This is a bad idea.
If you later move the import class to a different file, you may not be able to load old checkpoints. Did I know this anyway but still walk into this footgun? Yes.
The safe
but clunkier way is
torch.save(model.state_dict(), path)
.
Graph of the reconstruction loss over time:
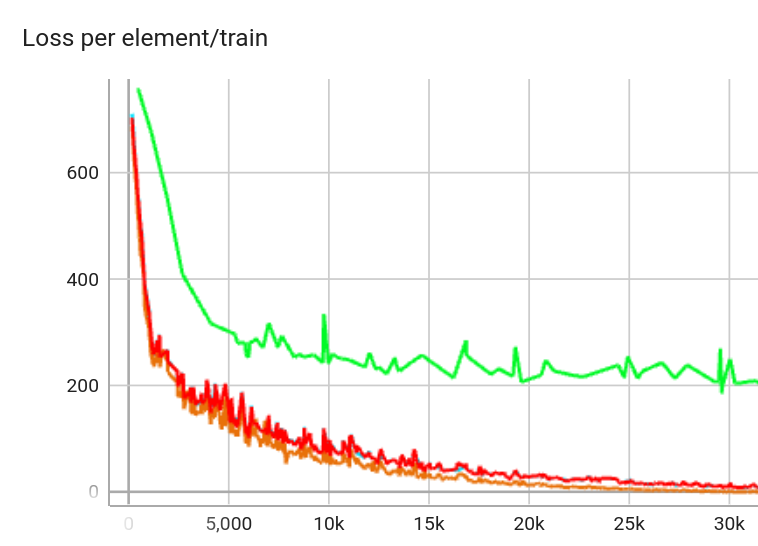
The blue curve is difficult to see because it’s almost perfectly covered by red.
All the curves converge to 0 loss, except for \(F=100\).
This makes sense: If you have to compress a vector in \( \mathbb{R}^{768} \) to a vector in \( \mathbb{R}^{100} \), you’re going to lose a lot of information that you can’t recover when decompressing.
Let’s zoom in on the x-axis:
Here we see that
\(F=767\)
isn’t actually converging to 0;
it gets stuck around loss = 0.1
.
We can also explain this: projecting from \( \mathbb{R}^{768} \) to \( \mathbb{R}^{767} \) (and back) loses just a little information.
Making it sparse
An autoencoder on its own is not so useful for interpreting the LLM. Just as the LLM learned incomprehensible ways of encoding text into 768 neural activations, the autoencoder will learn incomprehensible ways of encoding those neural activations into its \(F\) features.
Here’s how we encourage the autoencoder to make human-comprehensible features:
- First we make its job much easier by setting \(F\) to be much larger than 768. As we saw earlier, the autoencoder can’t perfectly preserve activations when \(F < 768\), but it does ok when \(F = 1000 \). Going forwards I’ll instead set \(F = 10000 \).
- Then we make its job harder by not letting it use very many features. We’d like to add a L0 penalty to the loss, i.e. we add the number of nonzero features to the loss. Because we need the loss to be differentiable, we instead use a L1 penalty: we add the sum of all the features to the loss. Since the features must be nonnegative because of the ReLU, this pushes most features to zero.
Q: I don’t see the motivation for these steps. Why is this expected to be make the features match up
to abstract ideas like “this text contains flying”?
A: It’ll be less mysterious once we go through some theory on how LLMs might think.
Q: Can I skip the theory and go to the experiments?
A: Sure, go to the next page.
Theory for how LLMs might think
(See Anthropic’s previous work “Toy Models of Superposition” for a longer explanation.)
Assumption 1: The LLM processes information in a sparse way. That is, it knows about thousands of human-understandable topics, but but an individual sentence only triggers a few of them at a time.
If the input text is “Mary had a little lamb”, the LLM’s internal representation
is something like
[contains_sheep = 0.5, female_character = 1, male_character = 0, contains_dog = 0, ...]
,
where the ...
is a long list of items that aren’t true about the story.
Let’s call these hypothesized items concepts,
to distinguish them from the \(F\) definitely real features
in the sparse autoencoder’s hidden layer. The end goal is to train the
autoencoder so that we can read off the concepts from the features.
These concepts
Assumption 2: The one-dimensional linear representation hypothesis—the concepts are in fact vectors in \(\mathbb{R}^{768}\), and the LLM stores information as a linear sum of near-perpendicular vectors.
When humans look at the activations, we just see
the sum of all concept vectors, and there’s
no easy way for humans to read that representation.
No one neuron of the 768 neurons is the contains_sheep
concept; it’s spread across all neurons.
It’s possible to fit exponentially more than 768 near-perpendicular vectors in \(\mathbb{R}^{768}\), so the LLM isn’t limited to 768 concepts.
Theory for how the sparse autoencoder should work
The sparse autoencoder has to transform the LLM activation \(l \in \mathbb{R}^{768} \) into a vector of features where only a few features are nonzero, and then recover \(l\). A plausible way to do this is:
- Use
encoder_linear
as 10000 linear probes. Each probe has two parts: \(a_i \in \mathbb{R}^{768} \) that points in the same direction as a concept vector, and a bias \(b_i \in \mathbb{R} \). Hence the ith autoencoder feature is \( f_i = a_i \cdot l + b_i \). Note that \(b_i \) is probably negative (all of this is theory), so if \( l \) doesn’t have a large component in the \(a_i\) direction, the ReLU will set the feature to 0. - Now only a few of the features are nonzero, satisfying the L1 penalty.
decoder_linear
just reversesencoder_linear
, mapping the ith feature back to the direction \(a_i \in \mathbb{R}^{768} \).- This reverse mapping isn’t perfect, since most features were set to 0 by the ReLU. But those features were associated with concepts that the LLM wasn’t thinking about (that’s the sparseness assumption). So not much of \(l\) is lost from the ReLU.
We don’t know that the autoencoder will use the above algorithm. But since this seems like the best algorithm, we hope that gradient descent will converge on it and also do the hard work of discovering the linear probes. (I’m hand-waving a lot here.)
The autoencoder has to reuse its features across all the examples in the training data. Hence the features must be synced with the concepts. So if we look at training examples where a certain feature was strongly activated, we should see a specific pattern.
Training a sparse autoencoder
Let’s get back to experiments. We want to train an autoencoder while minimizing the sum of the L2 reconstruction loss and the L1 sparsity penalty. The theory doesn’t tell us what their relative importance should be. So the loss function is really \[ \mathrm{loss} = \mathrm{L2\_reconstruction\_loss} + \lambda \cdot \mathrm{L1\_sparsity\_penalty} \]
where \( \lambda \) is an unknown hyperparameter. If \( \lambda \) is too small, we’ve just trained a non-sparse autoencoder. If it’s too large, gradient descent will prevent any feature from ever being nonzero.
Anthropic used \( \lambda = 5 \), but the optimal value could easily differ between implementations, e.g. if we used the mean L2 reconstruction loss instead of summing it, the L1 penalty would get a larger relative weight.
So let’s run a sweep over the L1 penalty strength \( \lambda \).
Which tensor do we apply the L1 penalty to? We can’t only apply it to the feature vector
\(f \in \mathbb{R}^{10000} \).
In that case the optimizer could cheat the L1 penalty by making all the features \(f_i\) small but nonzero,
while compensating by making the elements \(C_{ij}\) of decoder_linear
’s weight matrix large.
So instead we broadcast multiply \(f\) by \(C\) to get a list of 10000 feature vectors,
each one in \( \mathbb{R}^{768} \). Then we collapse each of those feature vectors into a single magnitude using
the L2 norm,
and then apply the L1 penalty to that list (call it scaled_features
) of 10000 numbers.
But look at those dimensions again.
We’ve created a tensor with dimensions (768, 10000)
. At 4 bytes per single-precision float,
that’s ~30 megabytes. We need one such tensor per token in the training example,
so we need 6 gigabytes when the example is 200 tokens long. All of this is batch size 1.
Luckily there’s an alternative route that avoids that large tensor:
- Apply the L2 norm to collapse \(C_{ij}\) into 10000 column magnitudes
- Multiply elementwise with \(f\)
- This is the same as
scaled_features
(importantly \(f_i \geq 0 \)) - Apply the L1 penalty
Tuning the L1 penalty
I trained 4 models:
- blue: \( \lambda = 0 \)
- green: \( \lambda = 5 \)
- orange: \( \lambda = 50 \)
- red: \( \lambda = 500 \)
Results of the sweep:
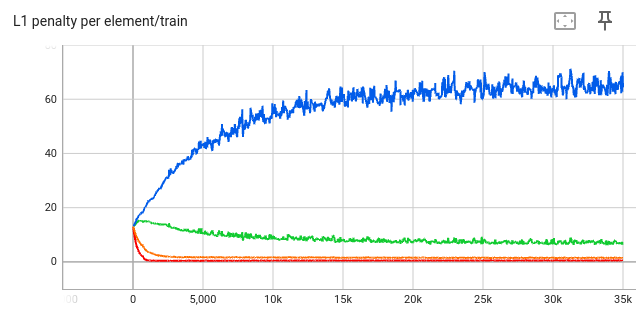
This is the L1 penalty before being multiplied by \(\lambda\)
As expected, a larger \(\lambda\) causes the L1 penalty to be smaller, since it’s more important in the loss function and hence the optimizer focuses on it more.
Conversely, a larger \(\lambda\) causes the L2 reconstruction loss to be worse:
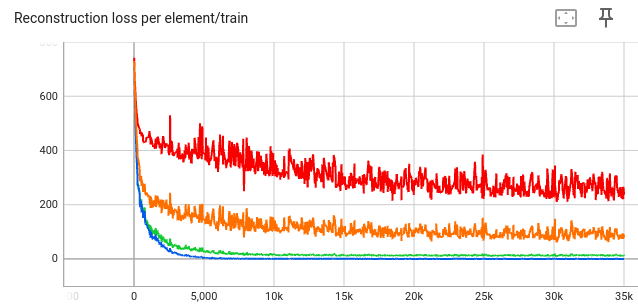
How do we choose which of these four models is the right one? Anthropic trained three sparse autoencoders, which each satsified this condition:
For all three SAEs, the average number of features active (i.e. with nonzero activations) on a given token was fewer than 300
So we can plot this value on the training set over time.
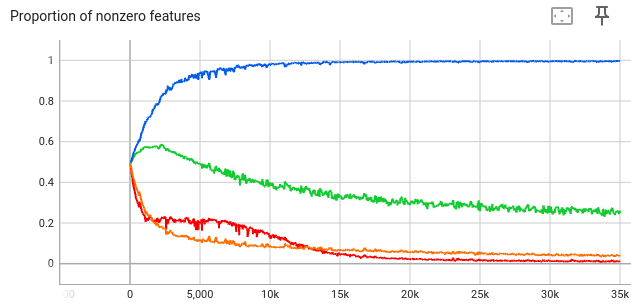
In particular, the values at step 35k are:
- For \( \lambda = 0 \): proportion = 0.9959, so there are 9959 active features
- This isn’t surprising—with no sparsity penalty, the autoencoder can use all the features to minimize reconstruction loss.
- For \( \lambda = 5 \): proportion = 0.2484, so there are 2484 active features
- For \( \lambda = 50 \): proportion = 0.03829, so there are ~383 active features
- For \( \lambda = 500 \): proportion = 0.01068, so there are ~107 active features
If we zoom in, we see that the proportion is still decreasing:
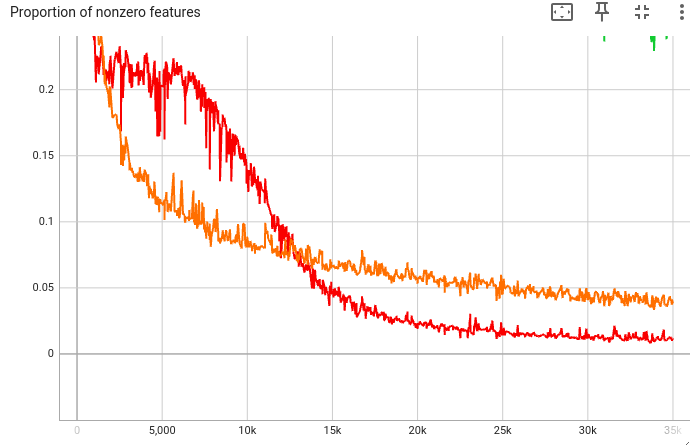
As a rough guide, I want this proportion to be under 300, but not too far—else the L1 penalty might be too strong. So let’s choose \( \lambda = 50 \) and train until step 105k, in the hope that the metric goes under 300.
(I’m choosing the number of steps so that it trains on a few hours on my laptop.)
And that’s what happens: At step 105k, there are roughly 156 active features:
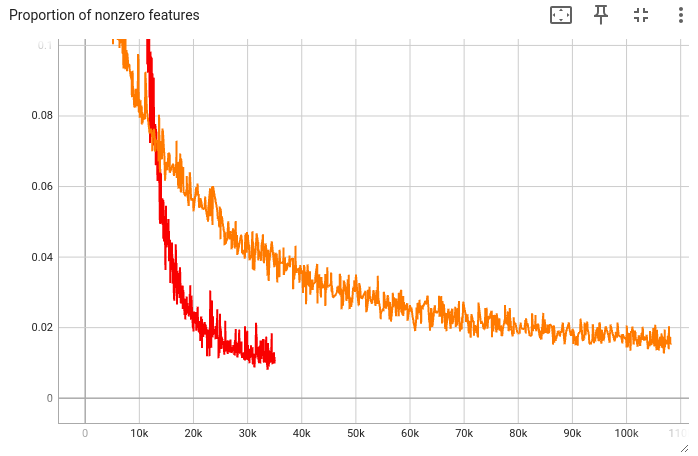
Step 105k is the checkpoint I’ll use for downstream experiments.
Comparing dataset size
At step 105k, the model has seen 2.29e7 tokens. Anthropic purposely left number of tokens off their graph, but in their previous work they trained for 8e9 tokens with much smaller models. (Note that I’m training on consecutive tokens, whereas they sampled and shuffled first.)
Anthropic says
As the compute budget increases, the optimal allocations of FLOPS to training steps and number of features both scale approximately as power laws. In general, the optimal number of features appears to scale somewhat more quickly than the optimal number of training steps
Since in the previous post their largest model had 1.3e5 parameters, while in “Scaling Monosemanticity” their largest model had 3.4e7 parameters, I estimate that the dataset scaled up also by roughly 100x, so I’d guess they trained their largest autoencoder on very roughly 1e12 tokens.
Manual feature examination
Now we have a trained autoencoder with a low reconstruction loss, and each feature is sparse—it only activates on a tiny fraction of input tokens.
But what we want is each input feature to be human-understandable and monosemantic. That is, each feature of the autoencoder should activate on input text with one narrow theme, like “cake/pie” or “numerical digits”.
To demonstrate that this actually happens, I’ll show the ten
examples from the roneneldan/TinyStories
validation set
where the feature activates most strongly.
I’ll use features 0, 1, and 2 (out of the 10000 available)
to avoid cherrypicking.
A more thorough investigation (e.g. this section of “Towards Monosemanticity”) would also check that the long tail of weaker activations shares the same theme.
How to read the examples
Each example is long, often > 200 words. Hence for readability I’ve hidden the examples and only display the excerpt where the feature activated most strongly. You can click on an example to expand it,
Suppose we’ve chosen a feature index (in this subsection 13) and a text from the validation set:
The sun was shining brightly and the birds were singing happily
We pass the text through the TinyStories language model (LM) and pass the LM’s residual state activation through the autoencoder. Thus we obtain a list telling us how strongly feature 13 activated on each token:
[
0.0,
0.0,
0.0,
0.0,
78.38675689697266,
0.0,
0.0,
0.0,
0.0,
0.0,
173.66055297851562,
]
To make this easier to read at a glance, I’ve used block elements to represent feature strength:
The ▁ sun ▁ was ▁ shining ▁ brightly ▄ and ▁ the ▁ birds ▁ were ▁ singing ▁ happily █
Here feature 13 activated once just after the LM saw “brightly”, and once after “happily”.
Sparse Autoencoder Features
Feature 0
My interpretation: This feature activates on the quotation mark when someone starts talking.
I’m not sure if the feature is aware of the context,
or if it’s specific to the character like “
.
GPT-4o’s interpretation: “Strong on exclamations and excitement”
(I sometimes disagree with GPT-4o’s interpretations—see the next page for a discussion.)
I think the weird artifacts like �
are caused by the tokenizer struggling
with quotation marks.
Example 1: he ▁ said ▁, ▁ � ▁� █€ ▁� ▁� ▁Wow ▁ thank ▁ you ▁ so ▁ much ▁!
Click to see all of example 1
Ben ▁ was ▁ a ▁ happy ▁ three ▁ year ▁ old ▁ boy ▁ who ▁ loved ▁ to ▁ explore ▁ his ▁ garden ▁. ▁ One ▁ day ▁, ▁ he ▁ noticed ▁ a ▁ little ▁ bee ▁ buzzing ▁ around ▁ the ▁ flowers ▁. ▁ He ▁ said ▁, ▁ � ▁� ▇€ ▁� ▁� ▁Hello ▁, ▁ little ▁ bee ▁. ▁â ▁€ ▁\n ▁\n ▁The ▁ bee ▁ flew ▁ up ▁ to ▁ him ▁ and ▁ buzz ▁ed ▁ in ▁ a ▁ friendly ▁ way ▁. ▁ Ben ▁ smiled ▁ and ▁ said ▁, ▁ � ▁� ▇€ ▁� ▁� ▁What ▁ are ▁ you ▁ doing ▁ here ▁? ▁â ▁€ ▁ ▁\n ▁\n ▁The ▁ bee ▁ flew ▁ away ▁ and ▁ returned ▁ a ▁ moment ▁ later ▁, ▁ carrying ▁ a ▁ drop ▁ of ▁ sweet ▁ honey ▁ in ▁ its ▁ tiny ▁ mouth ▁. ▁ Ben ▁â ▁€ ▁™ ▁s ▁ eyes ▁ lit ▁ up ▁ and ▁ he ▁ said ▁, ▁ � ▁� █€ ▁� ▁� ▁Wow ▁ thank ▁ you ▁ so ▁ much ▁! ▁ I ▁ love ▁ honey ▁! ▁â ▁€ ▁\n ▁\n ▁The ▁ bee ▁ flew ▁ away ▁ again ▁ and ▁ returned ▁ with ▁ some ▁ more ▁ honey ▁. ▁ Ben ▁ was ▁ filled ▁ with ▁ joy ▁ and ▁ said ▁, ▁ � ▁� ▇€ ▁� ▁� ▁Oh ▁, ▁ thank ▁ you ▁ so ▁ much ▁ lovely ▁ bee ▁! ▁ I ▁ love ▁ you ▁ too ▁! ▁â ▁€ ▁\n ▁\n ▁Just ▁ then ▁, ▁ a ▁ big ▁ dark ▁ cloud ▁ passed ▁ overhead ▁ and ▁ Ben ▁ felt ▁ a ▁ little ▁ bit ▁ miserable ▁. ▁ The ▁ bee ▁ flew ▁ up ▁ to ▁ him ▁ and ▁ buzz ▁ed ▁ in ▁ a ▁ comforting ▁ way ▁. ▁ Ben ▁ understood ▁ what ▁ the ▁ bee ▁ was ▁ trying ▁ to ▁ tell ▁ him ▁ and ▁ said ▁ � ▁� ▇€ ▁� ▁� ▁Thank ▁ you ▁, ▁ little ▁ bee ▁. ▁ I ▁ love ▁ you ▁. ▁ That ▁ helps ▁ me ▁ feel ▁ better ▁. ▁â ▁€ ▁\n ▁\n ▁The ▁ cloud ▁ soon ▁ passed ▁ and ▁ Ben ▁ and ▁ the ▁ bee ▁ went ▁ back ▁ to ▁ playing ▁ and ▁ collecting ▁ honey ▁. ▁ Ben ▁ knew ▁ he ▁ would ▁ always ▁ have ▁ the ▁ little ▁ bee ▁ with ▁ him ▁ in ▁ times ▁ of ▁ sadness ▁ and ▁ that ▁ made ▁ him ▁ feel ▁ very ▁ loved ▁ and ▁ happy ▁. ▁
Example 2: He ▁ smiled ▁, ▁ saying ▁, ▁ � ▁� █€ ▁� ▁� ▁Well ▁, ▁ I ▁â ▁€ ▁™ ▁m ▁ glad ▁ it ▁ wasn ▁â ▁€ ▁™ ▁t
Click to see all of example 2
Dog ▁ and ▁ Cat ▁ were ▁ best ▁ friends ▁ who ▁ did ▁ everything ▁ together ▁. ▁ Every ▁ day ▁ they ▁ would ▁ get ▁ up ▁ and ▁ play ▁ in ▁ the ▁ garden ▁. ▁\n ▁\n ▁One ▁ day ▁, ▁ Dog ▁ looked ▁ over ▁ and ▁ saw ▁ something ▁ disgusting ▁ on ▁ the ▁ ground ▁. ▁ He ▁ pointed ▁ and ▁ said ▁, ▁ � ▁� ▇€ ▁� ▁� ▁What ▁â ▁€ ▁™ ▁s ▁ that ▁? ▁â ▁€ ▁ Cat ▁ looked ▁ over ▁ and ▁ said ▁, ▁ � ▁� ▇€ ▁� ▁� ▁It ▁â ▁€ ▁™ ▁s ▁ gross ▁! ▁ Let ▁â ▁€ ▁™ ▁s ▁ not ▁ go ▁ near ▁ it ▁. ▁â ▁€ ▁\n ▁\n ▁The ▁ next ▁ day ▁ when ▁ Dog ▁ and ▁ Cat ▁ woke ▁ up ▁, ▁ they ▁ decided ▁ to ▁ play ▁ in ▁ the ▁ garden ▁ again ▁. ▁ But ▁ when ▁ they ▁ got ▁ there ▁, ▁ Dog ▁ saw ▁ the ▁ same ▁ disgusting ▁ thing ▁. ▁ He ▁ asked ▁ Cat ▁ again ▁, ▁ � ▁� ▇€ ▁� ▁� ▁What ▁â ▁€ ▁™ ▁s ▁ that ▁? ▁â ▁€ ▁ But ▁ this ▁ time ▁ Cat ▁ said ▁ nothing ▁. ▁\n ▁\n ▁Suddenly ▁, ▁ Dog ▁ felt ▁ angry ▁. ▁ He ▁ pointed ▁ at ▁ the ▁ thing ▁ and ▁ shouted ▁, ▁ � ▁� ▇€ ▁� ▁� ▁Why ▁ did ▁ you ▁ bring ▁ this ▁ here ▁!? ▁â ▁€ ▁ Cat ▁ looked ▁ embarrassed ▁ and ▁ said ▁, ▁ � ▁� ▇€ ▁� ▁� ▁I ▁ wanted ▁ to ▁ show ▁ you ▁ something ▁ I ▁ found ▁ yesterday ▁, ▁ but ▁ now ▁ it ▁â ▁€ ▁™ ▁s ▁ all ▁ gross ▁. ▁â ▁€ ▁\n ▁\n ▁Dog ▁ was ▁ surprised ▁ and ▁ relieved ▁. ▁ He ▁ smiled ▁, ▁ saying ▁, ▁ � ▁� █€ ▁� ▁� ▁Well ▁, ▁ I ▁â ▁€ ▁™ ▁m ▁ glad ▁ it ▁ wasn ▁â ▁€ ▁™ ▁t ▁ something ▁ bad ▁. ▁â ▁€ ▁ Dog ▁ and ▁ Cat ▁ hugged ▁ and ▁ went ▁ back ▁ to ▁ playing ▁. ▁
Example 3: Her ▁ mom ▁ smiled ▁ and ▁ said ▁, ▁ � ▁� █€ ▁� ▁� ▁Why ▁ don ▁â ▁€ ▁™ ▁t ▁ we ▁
Click to see all of example 3
M ▁agg ▁ie ▁ and ▁ her ▁ mom ▁ were ▁ out ▁ at ▁ the ▁ store ▁ shopping ▁. ▁ Maggie ▁â ▁€ ▁™ ▁s ▁ mom ▁ was ▁ looking ▁ at ▁ some ▁ fancy ▁ dresses ▁. ▁ She ▁ pointed ▁ to ▁ one ▁, ▁ saying ▁, ▁ � ▁� ▇€ ▁� ▁� ▁Look ▁ at ▁ this ▁ one ▁, ▁ Maggie ▁. ▁ Isn ▁â ▁€ ▁™ ▁t ▁ it ▁ nice ▁? ▁â ▁€ ▁\n ▁\n ▁â ▁€ ▁� ▁� ▁Yes ▁, ▁ Mom ▁my ▁, ▁ it ▁â ▁€ ▁™ ▁s ▁ pretty ▁, ▁â ▁€ ▁ Maggie ▁ said ▁. ▁ � ▁� ▇€ ▁� ▁� ▁It ▁â ▁€ ▁™ ▁s ▁ got ▁ a ▁ zip ▁, ▁ too ▁. ▁ I ▁ like ▁ it ▁. ▁â ▁€ ▁\n ▁\n ▁Her ▁ mom ▁ smiled ▁ and ▁ said ▁, ▁ � ▁� █€ ▁� ▁� ▁Why ▁ don ▁â ▁€ ▁™ ▁t ▁ we ▁ get ▁ it ▁ for ▁ you ▁? ▁â ▁€ ▁\n ▁\n ▁M ▁agg ▁ie ▁ was ▁ very ▁ excited ▁ and ▁ jumped ▁ up ▁ and ▁ down ▁. ▁ But ▁ then ▁ her ▁ mom ▁ noticed ▁ a ▁ sign ▁ that ▁ said ▁ � ▁� ▇€ ▁� ▁� ▁No ▁ Load ▁s ▁â ▁€ ▁. ▁ Maggie ▁ saw ▁ the ▁ sign ▁ too ▁ and ▁ asked ▁, ▁ � ▁� ▇€ ▁� ▁� ▁Mom ▁my ▁, ▁ why ▁ can ▁â ▁€ ▁™ ▁t ▁ we ▁ load ▁ the ▁ dress ▁? ▁â ▁€ ▁\n ▁\n ▁â ▁€ ▁� ▁� ▁It ▁ means ▁, ▁ Maggie ▁, ▁â ▁€ ▁ her ▁ mom ▁ said ▁ gently ▁, ▁ � ▁� ▇€ ▁� ▁� ▁that ▁ we ▁ can ▁â ▁€ ▁™ ▁t ▁ take ▁ it ▁ home ▁ with ▁ us ▁. ▁ We ▁â ▁€ ▁™ ▁ll ▁ have ▁ to ▁ leave ▁ it ▁ here ▁ today ▁. ▁ Maybe ▁ next ▁ time ▁ we ▁ can ▁ get ▁ it ▁. ▁â ▁€ ▁\n ▁\n ▁M ▁agg ▁ie ▁ was ▁ sad ▁ but ▁ she ▁ promised ▁ she ▁ wouldn ▁â ▁€ ▁™ ▁t ▁ forget ▁ the ▁ fancy ▁ dress ▁ and ▁ the ▁ zip ▁. ▁ She ▁ knew ▁ she ▁ would ▁ have ▁ to ▁ come ▁ back ▁ to ▁ the ▁ store ▁ soon ▁ so ▁ she ▁ could ▁ get ▁ the ▁ dress ▁ she ▁ wanted ▁. ▁
Example 4: The ▁ girl ▁ smiled ▁ and ▁ said ▁, ▁ � ▁� █€ ▁� ▁� ▁Okay ▁! ▁
Click to see all of example 4
Once ▁ upon ▁ a ▁ time ▁, ▁ a ▁ charming ▁ little ▁ girl ▁ went ▁ for ▁ a ▁ walk ▁ in ▁ the ▁ forest ▁. ▁ Suddenly ▁, ▁ she ▁ saw ▁ a ▁ snake ▁. ▁ She ▁ said ▁, ▁ � ▁� ▇€ ▁� ▁� ▁Oh ▁ no ▁! ▁â ▁€ ▁ and ▁ started ▁ to ▁ frown ▁. ▁\n ▁\n ▁But ▁ then ▁ the ▁ snake ▁ said ▁, ▁ � ▁� ▇€ ▁� ▁� ▁Don ▁â ▁€ ▁™ ▁t ▁ be ▁ scared ▁. ▁ I ▁â ▁€ ▁™ ▁m ▁ a ▁ friendly ▁ snake ▁! ▁â ▁€ ▁ The ▁ girl ▁ was ▁ surprised ▁, ▁ but ▁ she ▁ kept ▁ on ▁ frown ▁ing ▁. ▁ The ▁ snake ▁ said ▁, ▁ � ▁� ▇€ ▁� ▁� ▁You ▁ can ▁ trust ▁ me ▁. ▁ I ▁ promise ▁ not ▁ to ▁ hurt ▁ you ▁. ▁â ▁€ ▁ ▁\n ▁\n ▁The ▁ girl ▁ hesitated ▁, ▁ then ▁ the ▁ snake ▁ said ▁, ▁ � ▁� ▇€ ▁� ▁� ▁If ▁ you ▁ want ▁, ▁ I ▁ can ▁ show ▁ you ▁ around ▁ the ▁ forest ▁. ▁ It ▁â ▁€ ▁™ ▁s ▁ very ▁ beautiful ▁. ▁â ▁€ ▁ The ▁ girl ▁ smiled ▁ and ▁ said ▁, ▁ � ▁� █€ ▁� ▁� ▁Okay ▁! ▁â ▁€ ▁\n ▁\n ▁The ▁ girl ▁ and ▁ the ▁ snake ▁ went ▁ for ▁ a ▁ walk ▁ and ▁ had ▁ a ▁ wonderful ▁ time ▁. ▁ The ▁ snake ▁ showed ▁ her ▁ all ▁ the ▁ charming ▁ things ▁ in ▁ the ▁ forest ▁ and ▁ the ▁ girl ▁ was ▁ no ▁ longer ▁ afraid ▁. ▁\n ▁\n ▁At ▁ the ▁ end ▁ of ▁ the ▁ walk ▁, ▁ the ▁ girl ▁ said ▁, ▁ � ▁� ▇€ ▁� ▁� ▁Thank ▁ you ▁ for ▁ showing ▁ me ▁ around ▁ and ▁ making ▁ me ▁ feel ▁ less ▁ scared ▁. ▁â ▁€ ▁ The ▁ snake ▁ replied ▁, ▁ � ▁� ▇€ ▁� ▁� ▁It ▁ was ▁ my ▁ pleasure ▁. ▁ Come ▁ back ▁ and ▁ visit ▁ me ▁ anytime ▁. ▁â ▁€ ▁ The ▁ girl ▁ smiled ▁ and ▁ went ▁ happily ▁ on ▁ her ▁ way ▁. ▁
Example 5: and ▁ then ▁ he ▁ said ▁, ▁ � ▁� █€ ▁� ▁� ▁I ▁ think ▁ it ▁â ▁€ ▁™ ▁s ▁ one ▁
Click to see all of example 5
Once ▁ there ▁ was ▁ a ▁ little ▁ boy ▁ named ▁ Joe ▁. ▁ He ▁ was ▁ only ▁ three ▁ years ▁ old ▁ and ▁ loved ▁ to ▁ tell ▁ jokes ▁. ▁ One ▁ day ▁, ▁ Joe ▁ and ▁ his ▁ mom ▁ were ▁ walking ▁ home ▁ from ▁ the ▁ park ▁ when ▁ they ▁ saw ▁ a ▁ huge ▁ hot ▁ air ▁ balloon ▁. ▁ ▁\n ▁\n ▁â ▁€ ▁� ▁� ▁Look ▁, ▁ Mom ▁my ▁, ▁â ▁€ ▁ Joe ▁ said ▁, ▁ pointing ▁ at ▁ the ▁ balloon ▁. ▁ � ▁� ▇€ ▁� ▁� ▁It ▁â ▁€ ▁™ ▁s ▁ so ▁ big ▁. ▁â ▁€ ▁\n ▁\n ▁â ▁€ ▁� ▁� ▁Yes ▁, ▁ it ▁ certainly ▁ is ▁, ▁â ▁€ ▁ said ▁ his ▁ mom ▁. ▁ � ▁� ▇€ ▁� ▁� ▁Let ▁â ▁€ ▁™ ▁s ▁ guess ▁ how ▁ high ▁ it ▁ is ▁. ▁â ▁€ ▁\n ▁\n ▁Joe ▁ thought ▁ for ▁ a ▁ moment ▁ and ▁ then ▁ he ▁ said ▁, ▁ � ▁� █€ ▁� ▁� ▁I ▁ think ▁ it ▁â ▁€ ▁™ ▁s ▁ one ▁ thousand ▁ feet ▁. ▁â ▁€ ▁\n ▁\n ▁His ▁ mom ▁ laughed ▁. ▁ � ▁� ▇€ ▁� ▁� ▁That ▁â ▁€ ▁™ ▁s ▁ too ▁ high ▁, ▁ Joe ▁. ▁â ▁€ ▁\n ▁\n ▁Joe ▁ was ▁ disappointed ▁, ▁ but ▁ he ▁ wanted ▁ to ▁ prove ▁ he ▁ was ▁ right ▁. ▁ He ▁ remembered ▁ the ▁ joke ▁ he ▁ heard ▁ at ▁ the ▁ park ▁ earlier ▁. ▁\n ▁\n ▁â ▁€ ▁� ▁� ▁I ▁ know ▁ a ▁ joke ▁ that ▁ will ▁ tell ▁ us ▁, ▁â ▁€ ▁ he ▁ said ▁ excited ▁ly ▁. ▁ He ▁ rec ▁ited ▁ the ▁ joke ▁ he ▁ remembered ▁, ▁ making ▁ his ▁ mom ▁ laugh ▁. ▁\n ▁\n ▁Suddenly ▁, ▁ the ▁ balloon ▁ began ▁ to ▁ drift ▁ away ▁. ▁ It ▁ was ▁ getting ▁ higher ▁ and ▁ higher ▁. ▁ ▁\n ▁\n ▁Joe ▁ and ▁ his ▁ mom ▁ ran ▁ to ▁ catch ▁ up ▁ to ▁ it ▁, ▁ but ▁ the ▁ balloon ▁ moved ▁ too ▁ fast ▁. ▁ Joe ▁ looked ▁ at ▁ his ▁ mom ▁ and ▁ said ▁, ▁ � ▁� ▇€ ▁� ▁� ▁I ▁ guess ▁ I ▁ was ▁ right ▁ - ▁ it ▁ is ▁ one ▁ thousand ▁ feet ▁ high ▁! ▁â ▁€ ▁\n ▁\n ▁Joe ▁â ▁€ ▁™ ▁s ▁ mom ▁ smiled ▁ and ▁ said ▁, ▁ � ▁� ▇€ ▁� ▁� ▁You ▁ were ▁ right ▁, ▁ Joe ▁. ▁ That ▁â ▁€ ▁™ ▁s ▁ one ▁ hot ▁ joke ▁
Example 6: Mary ▁ smiled ▁ and ▁ said ▁, ▁ � ▁� █€ ▁� ▁� ▁We ▁ came ▁ to ▁ see ▁ the ▁ bird ▁
Click to see all of example 6
Mary ▁ and ▁ her ▁ big ▁ brother ▁ were ▁ playing ▁ in ▁ the ▁ park ▁ one ▁ sunny ▁ day ▁. ▁ As ▁ they ▁ ran ▁ around ▁, ▁ they ▁ spotted ▁ something ▁ shiny ▁ in ▁ the ▁ sky ▁. ▁ Mary ▁ said ▁, ▁ � ▁� ▇€ ▁� ▁� ▁What ▁ is ▁ that ▁? ▁â ▁€ ▁ Her ▁ brother ▁ said ▁, ▁ � ▁� ▇€ ▁� ▁� ▁It ▁â ▁€ ▁™ ▁s ▁ a ▁ bird ▁, ▁ but ▁ it ▁ isn ▁â ▁€ ▁™ ▁t ▁ flying ▁, ▁ it ▁ is ▁ diving ▁! ▁â ▁€ ▁ ▁\n ▁\n ▁Mary ▁ and ▁ her ▁ brother ▁ decided ▁ to ▁ follow ▁ the ▁ bird ▁ and ▁ see ▁ where ▁ it ▁ was ▁ going ▁. ▁ After ▁ a ▁ short ▁ walk ▁, ▁ they ▁ arrived ▁ at ▁ an ▁ old ▁, ▁ wooden ▁ house ▁ with ▁ a ▁ big ▁ attic ▁. ▁ The ▁ bird ▁ had ▁ d ▁ived ▁ into ▁ the ▁ attic ▁ and ▁ was ▁ now ▁ perched ▁ on ▁ a ▁ window ▁ sill ▁, ▁ pre ▁ening ▁ its ▁ feathers ▁. ▁ ▁\n ▁\n ▁When ▁ Mary ▁ and ▁ her ▁ brother ▁ knocked ▁ on ▁ the ▁ door ▁, ▁ an ▁ old ▁, ▁ deaf ▁ woman ▁ answered ▁. ▁ She ▁ wasn ▁â ▁€ ▁™ ▁t ▁ surprised ▁ to ▁ see ▁ the ▁ bird ▁, ▁ but ▁ was ▁ surprised ▁ to ▁ see ▁ the ▁ children ▁. ▁ She ▁ asked ▁, ▁ � ▁� ▇€ ▁� ▁� ▁What ▁ are ▁ you ▁ doing ▁ here ▁? ▁â ▁€ ▁ Mary ▁ smiled ▁ and ▁ said ▁, ▁ � ▁� █€ ▁� ▁� ▁We ▁ came ▁ to ▁ see ▁ the ▁ bird ▁ in ▁ your ▁ attic ▁! ▁â ▁€ ▁ ▁\n ▁\n ▁The ▁ old ▁ woman ▁ smiled ▁ and ▁ said ▁, ▁ � ▁� ▇€ ▁� ▁� ▁Come ▁ in ▁. ▁ The ▁ bird ▁ comes ▁ to ▁ visit ▁ me ▁ every ▁ day ▁. ▁ I ▁ can ▁â ▁€ ▁™ ▁t ▁ hear ▁ it ▁ singing ▁, ▁ but ▁ I ▁ love ▁ watching ▁ it ▁ dive ▁ down ▁ from ▁ the ▁ sky ▁. ▁â ▁€ ▁ Mary ▁ and ▁ her ▁ brother ▁ thanked ▁ the ▁ old ▁ woman ▁ and ▁ waved ▁ goodbye ▁ as ▁ they ▁ left ▁. ▁ ▁\n ▁\n ▁Mary ▁ and ▁ her ▁ brother ▁ had ▁ a ▁ lovely ▁ day ▁, ▁ thanks ▁ to ▁ the ▁ bird ▁ in ▁ the ▁ old ▁ woman ▁â ▁€ ▁™ ▁s ▁ attic ▁. ▁
Example 7: Mom ▁ said ▁, ▁ � ▁� █€ ▁� ▁� ▁I ▁ know ▁
Click to see all of example 7
Andy ▁ was ▁ playing ▁ in ▁ the ▁ park ▁. ▁ He ▁ saw ▁ a ▁ p ▁uddle ▁, ▁ and ▁ he ▁ was ▁ really ▁ excited ▁. ▁ He ▁ ran ▁ over ▁ to ▁ the ▁ p ▁uddle ▁ and ▁ said ▁ to ▁ Mom ▁, ▁ � ▁� ▇€ ▁� ▁� ▁Look ▁ at ▁ this ▁! ▁â ▁€ ▁ But ▁ Mom ▁ said ▁, ▁ � ▁� ▇€ ▁� ▁� ▁No ▁, ▁ Andy ▁, ▁ you ▁ can ▁â ▁€ ▁™ ▁t ▁ play ▁ here ▁. ▁ You ▁â ▁€ ▁™ ▁ll ▁ get ▁ all ▁ wet ▁. ▁â ▁€ ▁\n ▁\n ▁Andy ▁ was ▁ sad ▁, ▁ and ▁ he ▁ said ▁, ▁ � ▁� ▇€ ▁� ▁� ▁But ▁ I ▁ want ▁ to ▁ play ▁ in ▁ it ▁! ▁ Ple ▁ee ▁e ▁ase ▁! ▁â ▁€ ▁ Mom ▁ said ▁, ▁ � ▁� ▇€ ▁� ▁� ▁I ▁â ▁€ ▁™ ▁m ▁ sorry ▁, ▁ but ▁ you ▁ can ▁â ▁€ ▁™ ▁t ▁. ▁ It ▁â ▁€ ▁™ ▁s ▁ too ▁ dangerous ▁. ▁â ▁€ ▁\n ▁\n ▁Andy ▁ said ▁, ▁ � ▁� ▇€ ▁� ▁� ▁A ▁ww ▁ww ▁. ▁ That ▁â ▁€ ▁™ ▁s ▁ not ▁ fair ▁. ▁â ▁€ ▁ Mom ▁ said ▁, ▁ � ▁� █€ ▁� ▁� ▁I ▁ know ▁. ▁ I ▁â ▁€ ▁™ ▁m ▁ sorry ▁. ▁ You ▁ can ▁ play ▁ in ▁ the ▁ sandbox ▁ instead ▁. ▁ That ▁ will ▁ be ▁ safer ▁. ▁â ▁€ ▁\n ▁\n ▁Andy ▁ wasn ▁â ▁€ ▁™ ▁t ▁ sure ▁ if ▁ he ▁ wanted ▁ to ▁, ▁ but ▁ then ▁ he ▁ remembered ▁ how ▁ much ▁ fun ▁ it ▁ was ▁ to ▁ play ▁ in ▁ sand ▁. ▁ So ▁ he ▁ decided ▁ to ▁ go ▁ and ▁ play ▁ in ▁ the ▁ sandbox ▁ instead ▁. ▁ He ▁ was ▁ still ▁ a ▁ little ▁ bit ▁ sad ▁, ▁ but ▁ the ▁ thought ▁ of ▁ playing ▁ in ▁ the ▁ sand ▁ soon ▁ made ▁ him ▁ excited ▁ again ▁. ▁ ▁ He ▁ ran ▁ off ▁ to ▁ the ▁ sandbox ▁, ▁ ready ▁ to ▁ have ▁ some ▁ fun ▁. ▁
Example 8: T ▁oby ▁ nodded ▁ in ▁ understanding ▁ and ▁ said ▁, ▁ � ▁� █€ ▁� ▁� ▁Be ▁ brave ▁
Click to see all of example 8
T ▁oby ▁ was ▁ a ▁ young ▁ boy ▁, ▁ he ▁ was ▁ very ▁ curious ▁. ▁ One ▁ day ▁ as ▁ he ▁ was ▁ playing ▁ in ▁ the ▁ park ▁ he ▁ noticed ▁ a ▁ cricket ▁ ch ▁ir ▁ping ▁ loudly ▁. ▁ Toby ▁ wondered ▁ what ▁ the ▁ cricket ▁ was ▁ saying ▁. ▁ He ▁ also ▁ noticed ▁ that ▁ the ▁ cricket ▁ looked ▁ quite ▁ nervous ▁. ▁ Toby ▁ had ▁ many ▁ questions ▁ so ▁ he ▁ decided ▁ to ▁ ask ▁. ▁\n ▁\n ▁â ▁€ ▁� ▁� ▁Hello ▁ little ▁ cricket ▁,“ ▁ Toby ▁ said ▁. ▁\n ▁\n ▁The ▁ cricket ▁ stopped ▁ ch ▁ir ▁ping ▁, ▁ looked ▁ around ▁ and ▁ said ▁ � ▁� ▇€ ▁� ▁� ▁yes ▁, ▁ what ▁ is ▁ it ▁? ▁â ▁€ ▁ ▁\n ▁\n ▁â ▁€ ▁� ▁� ▁Why ▁ do ▁ you ▁ look ▁ so ▁ nervous ▁? ▁â ▁€ ▁ Toby ▁ asked ▁. ▁\n ▁\n ▁The ▁ cricket ▁ took ▁ a ▁ deep ▁ breath ▁ and ▁ said ▁, ▁ � ▁� ▇€ ▁� ▁� ▁I ▁ need ▁ to ▁ go ▁ somewhere ▁ but ▁ I ▁’m ▁ scared ▁ to ▁ leave ▁.“ ▁\n ▁\n ▁T ▁oby ▁ nodded ▁ in ▁ understanding ▁ and ▁ said ▁, ▁ � ▁� █€ ▁� ▁� ▁Be ▁ brave ▁ little ▁ cricket ▁! ▁ I ▁’m ▁ sure ▁ you ▁’ll ▁ make ▁ it ▁ there ▁ safe ▁. ▁â ▁€ ▁\n ▁\n ▁The ▁ cricket ▁ smiled ▁ weak ▁ly ▁ and ▁ said ▁, ▁ � ▁� ▇€ ▁� ▁� ▁Thank ▁ you ▁! ▁ Now ▁ I ▁ just ▁ need ▁ to ▁ find ▁ the ▁ way ▁. ▁â ▁€ ▁ ▁\n ▁\n ▁T ▁oby ▁ smiled ▁ and ▁ said ▁, ▁ � ▁� ▇€ ▁� ▁� ▁Maybe ▁ I ▁ can ▁ help ▁ you ▁. ▁ Would ▁ you ▁ like ▁ that ▁? ▁â ▁€ ▁ ▁\n ▁\n ▁The ▁ cricket ▁ nodded ▁, ▁ � ▁� ▇€ ▁� ▁� ▁Yes ▁, ▁ I ▁ would ▁ appreciate ▁ it ▁ very ▁ much ▁! ▁â ▁€ ▁\n ▁\n ▁So ▁ Toby ▁ and ▁ the ▁ cricket ▁ began ▁ walking ▁ together ▁. ▁ Toby ▁ was ▁ filled ▁ with ▁ wonder ▁ and ▁ admiration ▁ for ▁ the ▁ brave ▁ little ▁ cricket ▁. ▁ With ▁ Toby ▁ as ▁ his ▁ guide ▁, ▁ the ▁ cricket ▁ was ▁ no ▁ longer ▁ nervous ▁ - ▁ he ▁ was ▁ feeling ▁ brave ▁ and ▁ confident ▁. ▁ Toby ▁ was ▁ happy ▁ he ▁ could ▁ help ▁ the ▁ cricket ▁ on ▁ his ▁ journey ▁. ▁
Example 9: He ▁ said ▁, ▁ � ▁� █€ ▁� ▁� ▁No ▁, ▁ let ▁â ▁€ ▁™ ▁s ▁ go ▁
Click to see all of example 9
Once ▁ upon ▁ a ▁ time ▁, ▁ there ▁ were ▁ two ▁ children ▁. ▁ One ▁ child ▁ was ▁ clever ▁, ▁ the ▁ other ▁ was ▁ very ▁ foolish ▁. ▁ They ▁ were ▁ walking ▁ along ▁ the ▁ path ▁ to ▁ school ▁ when ▁ they ▁ passed ▁ by ▁ a ▁ garden ▁. ▁ The ▁ clever ▁ child ▁ said ▁ to ▁ the ▁ foolish ▁ one ▁, ▁ � ▁� ▇€ ▁� ▁� ▁We ▁ should ▁ poke ▁ that ▁ garden ▁. ▁â ▁€ ▁ ▁\n ▁\n ▁But ▁ the ▁ foolish ▁ one ▁ said ▁, ▁ � ▁� ▇€ ▁� ▁� ▁No ▁, ▁ don ▁â ▁€ ▁™ ▁t ▁ be ▁ silly ▁. ▁ That ▁â ▁€ ▁™ ▁s ▁ not ▁ a ▁ good ▁ thing ▁ to ▁ do ▁. ▁ We ▁ should ▁ focus ▁ on ▁ the ▁ math ▁ lesson ▁. ▁â ▁€ ▁ ▁\n ▁\n ▁The ▁ clever ▁ child ▁ laughed ▁ and ▁ said ▁, ▁ � ▁� ▇€ ▁� ▁� ▁Don ▁â ▁€ ▁™ ▁t ▁ worry ▁, ▁ I ▁ just ▁ thought ▁ it ▁ would ▁ be ▁ fun ▁. ▁â ▁€ ▁ ▁\n ▁\n ▁Still ▁, ▁ the ▁ foolish ▁ one ▁ didn ▁â ▁€ ▁™ ▁t ▁ bud ▁ge ▁. ▁ He ▁ said ▁, ▁ � ▁� █€ ▁� ▁� ▁No ▁, ▁ let ▁â ▁€ ▁™ ▁s ▁ go ▁ to ▁ school ▁ now ▁. ▁ Math ▁ is ▁ more ▁ important ▁. ▁â ▁€ ▁ ▁\n ▁\n ▁So ▁ the ▁ two ▁ children ▁ continued ▁ on ▁ their ▁ way ▁ to ▁ school ▁. ▁ But ▁ when ▁ they ▁ got ▁ there ▁, ▁ they ▁ were ▁ surprised ▁ to ▁ see ▁ their ▁ teacher ▁ with ▁ a ▁ garden ▁ fork ▁ in ▁ his ▁ hand ▁. ▁ He ▁ was ▁ mad ▁ and ▁ said ▁ to ▁ the ▁ two ▁ children ▁, ▁ � ▁� ▇€ ▁� ▁� ▁Why ▁ did ▁ you ▁ poke ▁ my ▁ garden ▁?! ▁â ▁€ ▁ ▁\n ▁\n ▁The ▁ clever ▁ child ▁ smiled ▁ and ▁ said ▁, ▁ � ▁� ▇€ ▁� ▁� ▁I ▁ told ▁ the ▁ foolish ▁ one ▁ that ▁ it ▁ would ▁ be ▁ fun ▁. ▁ He ▁ didn ▁â ▁€ ▁™ ▁t ▁ want ▁ to ▁ do ▁ it ▁, ▁ so ▁ I ▁ did ▁ it ▁ myself ▁. ▁â ▁€ ▁ ▁\n ▁\n ▁The ▁ teacher ▁ shook ▁ his ▁ head ▁ and ▁ said ▁, ▁ � ▁� ▇€ ▁� ▁� ▁That ▁ was ▁ foolish ▁ of ▁ you ▁. ▁ Next ▁ time ▁, ▁ focus ▁ on ▁ math ▁ instead ▁. ▁â ▁€ ▁ ▁\n ▁\n ▁The ▁ two ▁ children ▁ learned ▁ their ▁ lesson ▁
Example 10: Anna ▁ stepped ▁ forward ▁ and ▁ said ▁ in ▁ a ▁ friendly ▁ voice ▁, ▁ � ▁� █€ ▁� ▁� ▁Hello ▁ there ▁!
Click to see all of example 10
Once ▁ upon ▁ a ▁ time ▁, ▁ there ▁ was ▁ an ▁ ordinary ▁ house ▁. ▁ Inside ▁ the ▁ house ▁ lived ▁ two ▁ children ▁, ▁ Mike ▁ and ▁ Anna ▁. ▁\n ▁\n ▁One ▁ day ▁, ▁ Mike ▁ said ▁ to ▁ Anna ▁, ▁ � ▁� ▇€ ▁� ▁� ▁Let ▁â ▁€ ▁™ ▁s ▁ go ▁ on ▁ an ▁ adventure ▁. ▁ Let ▁â ▁€ ▁™ ▁s ▁ bring ▁ our ▁ toys ▁ and ▁ explore ▁ the ▁ wild ▁! ▁â ▁€ ▁ Anna ▁ smiled ▁ and ▁ happily ▁ agreed ▁ to ▁ go ▁ on ▁ an ▁ adventure ▁. ▁\n ▁\n ▁So ▁, ▁ Mike ▁ and ▁ Anna ▁ grabbed ▁ their ▁ toys ▁ and ▁ went ▁ outside ▁. ▁ ▁\n ▁\n ▁The ▁ wild ▁ was ▁ filled ▁ with ▁ tall ▁ trees ▁, ▁ colourful ▁ flowers ▁ and ▁ butterflies ▁ flying ▁ around ▁. ▁ It ▁ was ▁ a ▁ beautiful ▁ sight ▁. ▁ Anna ▁ gigg ▁led ▁ with ▁ delight ▁, ▁ � ▁� ▇€ ▁� ▁� ▁Look ▁, ▁ Mike ▁! ▁â ▁€ ▁\n ▁\n ▁Eventually ▁, ▁ Mike ▁ and ▁ Anna ▁ noticed ▁ a ▁ tiny ▁, ▁ furry ▁ creature ▁. ▁ It ▁ was ▁ the ▁ cut ▁est ▁ thing ▁ they ▁ had ▁ ever ▁ seen ▁. ▁ Mike ▁ said ▁ gently ▁, ▁ � ▁� ▇€ ▁� ▁� ▁Let ▁â ▁€ ▁™ ▁s ▁ introduce ▁ ourselves ▁. ▁ Don ▁â ▁€ ▁™ ▁t ▁ be ▁ scared ▁. ▁â ▁€ ▁ ▁\n ▁\n ▁Anna ▁ stepped ▁ forward ▁ and ▁ said ▁ in ▁ a ▁ friendly ▁ voice ▁, ▁ � ▁� █€ ▁� ▁� ▁Hello ▁ there ▁! ▁ We ▁â ▁€ ▁™ ▁re ▁ Mike ▁ and ▁ Anna ▁. ▁ It ▁â ▁€ ▁™ ▁s ▁ nice ▁ to ▁ meet ▁ you ▁! ▁â ▁€ ▁\n ▁\n ▁The ▁ tiny ▁ creature ▁ smiled ▁ and ▁ said ▁, ▁ � ▁� ▇€ ▁� ▁� ▁Hi ▁ there ▁! ▁ My ▁ name ▁ is ▁ Brown ▁ie ▁. ▁ Welcome ▁ to ▁ the ▁ wild ▁! ▁â ▁€ ▁ ▁\n ▁\n ▁Mike ▁ and ▁ Anna ▁ smiled ▁ and ▁ they ▁ all ▁ became ▁ great ▁ friends ▁. ▁ From ▁ that ▁ day ▁ onward ▁, ▁ they ▁ would ▁ often ▁ explore ▁ the ▁ wild ▁ together ▁. ▁
Feature 1
My interpretation: This feature activates on a list of concrete nouns, almost always in the context of characters wondering about this list or playing pretend with the list.
GPT-4o’s interpretation: “Strong highlights for imaginary roles or objects.”
Example 1: He ▁ pret ▁ends ▁ it ▁ is ▁ a ▂ plane ▂ or ▃ a █ bird ▆ or ▄ a ▇ rocket ▅. ▁
Click to see all of example 1
Tom ▁ and ▁ Anna ▁ are ▁ playing ▁ in ▁ the ▁ backyard ▁. ▁ They ▁ see ▁ a ▁ big ▁ wire ▁ on ▁ the ▁ ground ▁. ▁ It ▁ is ▁ long ▁ and ▁ shiny ▁ and ▁ has ▁ a ▁ hook ▁ at ▁ the ▁ end ▁. ▁\n ▁\n ▁“ ▁Look ▁, ▁ a ▁ wire ▁!“ ▁ Tom ▁ says ▁. ▁ “ ▁Let ▁’s ▁ play ▁ with ▁ it ▁!“ ▁\n ▁\n ▁“ ▁OK ▁!“ ▁ Anna ▁ says ▁. ▁ “ ▁What ▁ can ▁ we ▁ do ▁ with ▁ it ▁?“ ▁\n ▁\n ▁Tom ▁ thinks ▁ for ▁ a ▁ moment ▁. ▁ He ▁ sees ▁ a ▁ big ▁ rock ▁ near ▁ the ▁ fence ▁. ▁ He ▁ has ▁ an ▁ idea ▁. ▁\n ▁\n ▁“ ▁Let ▁’s ▁ weigh ▁ the ▁ rock ▁!“ ▁ he ▁ says ▁. ▁ “ ▁We ▁ can ▁ use ▁ the ▁ wire ▁ as ▁ a ▁ scale ▁!“ ▁\n ▁\n ▁He ▁ picks ▁ up ▁ the ▁ wire ▁ and ▁ wraps ▁ it ▁ around ▁ the ▁ rock ▁. ▁ He ▁ holds ▁ the ▁ hook ▁ in ▁ his ▁ hand ▁ and ▁ lifts ▁ the ▁ rock ▁. ▁\n ▁\n ▁“ ▁Wow ▁, ▁ it ▁’s ▁ heavy ▁!“ ▁ he ▁ says ▁. ▁ “ ▁Can ▁ you ▁ guess ▁ how ▁ much ▁ it ▁ weighs ▁?“ ▁\n ▁\n ▁Anna ▁ looks ▁ at ▁ the ▁ rock ▁. ▁ She ▁ does ▁ not ▁ know ▁ how ▁ to ▁ weigh ▁ things ▁. ▁ She ▁ does ▁ not ▁ know ▁ what ▁ a ▁ scale ▁ is ▁. ▁ She ▁ does ▁ not ▁ know ▁ what ▁ a ▁ pound ▁ or ▁ a ▃ kil ▁o ▁ is ▁. ▁ She ▁ only ▁ knows ▁ big ▁ and ▁ small ▁, ▁ light ▁ and ▁ heavy ▁. ▁\n ▁\n ▁“ ▁I ▁ don ▁’t ▁ know ▁,“ ▁ she ▁ says ▁. ▁ “ ▁Maybe ▁ it ▁ weighs ▁… ▁ a ▁ lot ▁?“ ▁\n ▁\n ▁Tom ▁ laughs ▁. ▁ He ▁ thinks ▁ Anna ▁ is ▁ funny ▁. ▁ He ▁ likes ▁ playing ▁ with ▁ her ▁. ▁\n ▁\n ▁“ ▁Maybe ▁ it ▁ does ▁,“ ▁ he ▁ says ▁. ▁ “ ▁But ▁ I ▁ have ▁ a ▁ better ▁ idea ▁. ▁ Let ▁’s ▁ swing ▁ the ▁ rock ▁!“ ▁\n ▁\n ▁He ▁ swings ▁ the ▁ rock ▁ with ▁ the ▁ wire ▁. ▁ He ▁ makes ▁ it ▁ go ▁ up ▁ and ▁ down ▁, ▂ left ▂ and ▃ right ▄. ▁ He ▁ pret ▁ends ▁ it ▁ is ▁ a ▂ plane ▂ or ▃ a █ bird ▆ or ▄ a ▇ rocket ▅. ▁\n ▁\n ▁Anna ▁ cl ▁aps ▁ her ▁ hands ▁. ▁ She ▁ thinks ▁ Tom ▁ is ▁ clever ▁. ▁ She ▁ likes ▁ swinging ▁ the ▁ rock ▁. ▁\n ▁\n ▁“ ▁Y ▁ay ▁, ▁ this ▁ is ▁ fun ▁!“ ▁ she ▁ says ▁. ▁ “ ▁Let ▁’s ▁ swing ▁ it ▁ higher ▁!“ ▁\n ▁\n ▁They ▁ swing ▁ the ▁ rock ▁ higher ▁ and ▁ higher ▁. ▁ They ▁ do ▁ not ▁ see ▁ the ▁ unknown ▁ man ▁ who ▁ is ▁ watching ▁ them ▁ from ▁ the ▁ other ▁ side ▁ of ▁ the ▁ fence ▁. ▁ He ▁ is ▁ angry ▁. ▁ He ▁ does ▁ not ▁ like ▁ children ▁. ▁ He ▁ does ▁ not ▁ like ▁ noise ▁. ▁ He ▁ does ▁ not ▁ like ▁ his ▁ wire ▁ being ▁ used ▁ as ▁ a ▁ toy ▂. ▁\n ▁\n ▁He ▁ shouts ▁ at ▁ them ▁. ▁ He ▁ tells ▁ them ▁ to ▁ stop ▁. ▁ He ▁ tells ▁ them ▁ to ▁ give ▁ him ▁ back ▁ his ▁ wire ▁. ▁ He ▁ tells ▁ them ▁ to ▁ go ▁ away ▁. ▁\n ▁\n ▁Tom ▁ and ▁ Anna ▁ are ▁ scared ▁. ▁ They ▁ do ▁ not ▁ know ▁ the ▁ man ▁. ▁ They ▁ do ▁ not ▁ know ▁ why ▁ he ▁ is ▁ mad ▁. ▁ They ▁ do ▁ not ▁ know ▁ what ▁ to ▁ do ▁. ▁\n ▁\n ▁They ▁ drop ▁ the ▁ wire ▁ and ▁ the ▁ rock ▁. ▁ They ▁ run ▁ to ▁ the ▁ house ▁. ▁ They ▁ tell ▁ their ▁ mom ▁ what ▁ happened ▁. ▁\n ▁\n ▁Their ▁ mom ▁ hugs ▁ them ▁. ▁ She ▁ tells ▁ them ▁ they ▁ are ▁ safe ▁. ▁ She ▁ tells ▁ them ▁ they ▁ did ▁ nothing ▁ wrong ▁. ▁ She ▁ tells ▁ them ▁ the ▁ wire ▁ belongs ▁ to ▁ the ▁ electric ▁ company ▁. ▁ She ▁ tells ▁ them ▁ the ▁ man ▁ is ▁ a ▁ worker ▁ who ▁ is ▁ fixing ▁ the ▁ power ▁ lines ▁. ▁ She ▁ tells ▁ them ▁ he ▁ should ▁ not ▁ have ▁ yelled ▁ at ▁ them ▁. ▁ She ▁ tells ▁ them ▁ he ▁ should ▁ have ▁ been ▁ nicer ▁. ▁\n ▁\n ▁Tom ▁ and ▁ Anna ▁ feel ▁ better ▁. ▁ They ▁ understand ▁ now ▁. ▁ They ▁ are ▁ not ▁ afraid ▁ anymore ▁. ▁\n ▁\n ▁They ▁ go ▁ back ▁ to ▁ the ▁ backyard ▁. ▁ They ▁ find ▁ a ▁ new ▁ toy ▁ to ▁ play ▁ with ▁. ▁ They ▁ have ▁ fun ▁. ▁ They ▁ are ▁ happy ▁. ▁
Example 2: and ▁ pretend ▁ it ▁ was ▁ a ▂ spaceship ▃ or ▅ a █ tunnel ▅. ▁
Click to see all of example 2
Tom ▁ and ▁ Lily ▁ were ▁ playing ▁ with ▁ a ▁ big ▁ tube ▁ in ▁ the ▁ garden ▁. ▁ The ▁ tube ▁ was ▁ white ▁ and ▁ had ▁ a ▁ red ▁ cap ▁. ▁ They ▁ liked ▁ to ▁ crawl ▁ inside ▁ the ▁ tube ▁ and ▁ pretend ▁ it ▁ was ▁ a ▂ spaceship ▃ or ▅ a █ tunnel ▅. ▁\n ▁\n ▁“ ▁Let ▁’s ▁ go ▁ to ▁ the ▁ moon ▁!“ ▁ Tom ▁ said ▁, ▁ putting ▁ the ▁ cap ▁ on ▁ one ▁ end ▁ of ▁ the ▁ tube ▁. ▁\n ▁\n ▁“ ▁OK ▁!“ ▁ Lily ▁ said ▁, ▁ following ▁ him ▁ inside ▁. ▁ They ▁ made ▁ noises ▁ like ▁ rockets ▁ and ▁ stars ▁. ▁\n ▁\n ▁But ▁ they ▁ did ▁ not ▁ know ▁ that ▁ the ▁ tube ▁ was ▁ not ▁ a ▁ toy ▁. ▁ It ▁ was ▁ a ▁ special ▁ tube ▁ that ▁ their ▁ dad ▁ used ▁ for ▁ his ▁ work ▁. ▁ The ▁ tube ▁ had ▁ a ▁ button ▁ that ▁ could ▁ make ▁ things ▁ shrink ▁ or ▁ grow ▁. ▁\n ▁\n ▁When ▁ Tom ▁ and ▁ Lily ▁ were ▁ inside ▁, ▁ the ▁ button ▁ got ▁ pressed ▁ by ▁ a ▁ rock ▁. ▁ The ▁ tube ▁ started ▁ to ▁ make ▁ a ▁ loud ▁ sound ▁ and ▁ a ▁ bright ▁ light ▁. ▁ Tom ▁ and ▁ Lily ▁ felt ▁ very ▁ scared ▁ and ▁ tried ▁ to ▁ get ▁ out ▁. ▁\n ▁\n ▁“ ▁Help ▁! ▁ Help ▁!“ ▁ they ▁ shouted ▁. ▁ “ ▁We ▁ are ▁ stuck ▁!“ ▁\n ▁\n ▁Their ▁ dad ▁ heard ▁ them ▁ and ▁ ran ▁ to ▁ the ▁ garden ▁. ▁ He ▁ saw ▁ the ▁ tube ▁ and ▁ realized ▁ what ▁ had ▁ happened ▁. ▁ He ▁ tried ▁ to ▁ press ▁ the ▁ button ▁ again ▁, ▁ but ▁ it ▁ was ▁ broken ▁. ▁ He ▁ could ▁ not ▁ make ▁ the ▁ tube ▁ stop ▁. ▁\n ▁\n ▁He ▁ looked ▁ inside ▁ the ▁ tube ▁ and ▁ saw ▁ Tom ▁ and ▁ Lily ▁. ▁ They ▁ were ▁ very ▁ small ▁, ▁ like ▁ ants ▁. ▁ They ▁ looked ▁ at ▁ him ▁ with ▁ big ▁ eyes ▁ and ▁ cried ▁. ▁\n ▁\n ▁“ ▁Dad ▁! ▁ Dad ▁! ▁ Save ▁ us ▁!“ ▁ they ▁ said ▁. ▁\n ▁\n ▁But ▁ their ▁ dad ▁ could ▁ not ▁ hear ▁ them ▁. ▁ He ▁ could ▁ not ▁ reach ▁ them ▁. ▁ He ▁ could ▁ not ▁ help ▁ them ▁. ▁\n ▁\n ▁He ▁ felt ▁ very ▁ sad ▁ and ▁ sorry ▁. ▁ He ▁ had ▁ lost ▁ his ▁ children ▁. ▁ They ▁ had ▁ shrunk ▁ in ▁ the ▁ tube ▁. ▁
Example 3: and ▁ pretend ▁ it ▁ is ▁ a ▂ spaceship ▃, ▃ a ▆ castle ▄ or ▆ a █ dragon ▄. ▁
Click to see all of example 3
L ▁ily ▁ and ▁ Max ▁ are ▁ best ▁ friends ▁. ▁ They ▁ like ▁ to ▁ play ▁ in ▁ the ▁ park ▁ every ▁ day ▁. ▁ They ▁ have ▁ a ▁ lot ▁ of ▁ fun ▁ on ▁ the ▁ swings ▁, ▁ the ▁ slide ▁ and ▁ the ▃ sees ▁aw ▁. ▁ But ▁ their ▁ favorite ▁ thing ▁ is ▁ the ▁ big ▁ rock ▁. ▁\n ▁\n ▁The ▁ big ▁ rock ▁ is ▁ incredible ▁. ▁ It ▁ is ▁ very ▁ big ▁ and ▁ very ▁ smooth ▁. ▁ It ▁ has ▁ many ▁ colors ▁ and ▁ shapes ▁ on ▁ it ▁. ▁ Lily ▁ and ▁ Max ▁ like ▁ to ▁ climb ▁ on ▁ it ▁, ▁ sit ▁ on ▁ it ▁ and ▁ pretend ▁ it ▁ is ▁ a ▂ spaceship ▃, ▃ a ▆ castle ▄ or ▆ a █ dragon ▄. ▁\n ▁\n ▁One ▁ day ▁, ▁ they ▁ find ▁ a ▁ new ▁ game ▁. ▁ They ▁ sit ▁ on ▁ the ▁ rock ▁ and ▁ hold ▁ hands ▁. ▁ Then ▁ they ▁ lean ▁ back ▁ and ▁ forth ▁, ▁ making ▁ the ▁ rock ▁ move ▁ a ▁ little ▁. ▁ They ▁ g ▁iggle ▁ and ▁ shout ▁, ▁ “ ▁We ▁ are ▁ rocking ▁ the ▁ rock ▁! ▁ We ▁ are ▁ rocking ▁ the ▁ rock ▁!“ ▁\n ▁\n ▁They ▁ rock ▁ the ▁ rock ▁ faster ▁ and ▁ faster ▁, ▁ feeling ▁ the ▁ wind ▁ in ▁ their ▁ hair ▁ and ▁ the ▁ sun ▁ on ▁ their ▁ faces ▁. ▁ They ▁ are ▁ very ▁ happy ▁ and ▁ their ▁ smiles ▁ are ▁ very ▁ big ▁. ▁\n ▁\n ▁“ ▁Look ▁ at ▁ us ▁!“ ▁ Lily ▁ says ▁. ▁ “ ▁We ▁ are ▁ the ▁ best ▁ rock ▁ers ▁ in ▁ the ▁ park ▁!“ ▁\n ▁\n ▁“ ▁Yes ▁, ▁ we ▁ are ▁!“ ▁ Max ▁ says ▁. ▁ “ ▁We ▁ are ▁ the ▁ best ▁ friends ▁ in ▁ the ▁ world ▁!“ ▁\n ▁\n ▁They ▁ rock ▁ the ▁ rock ▁ until ▁ they ▁ are ▁ tired ▁. ▁ Then ▁ they ▁ hug ▁ each ▁ other ▁ and ▁ say ▁, ▁ “ ▁Thank ▁ you ▁ for ▁ rocking ▁ with ▁ me ▁. ▁ You ▁ are ▁ incredible ▁!“ ▁\n ▁\n ▁They ▁ get ▁ off ▁ the ▁ rock ▁ and ▁ run ▁ to ▁ their ▁ moms ▁, ▁ who ▁ are ▁ waiting ▁ for ▁ them ▁ with ▁ juice ▁ and ▁ cookies ▂. ▁ They ▁ tell ▁ them ▁ about ▁ their ▁ game ▁ and ▁ how ▁ much ▁ fun ▁ they ▁ had ▁. ▁ Their ▁ moms ▁ smile ▁ and ▁ say ▁, ▁ “ ▁We ▁ are ▁ glad ▁ you ▁ had ▁ a ▁ good ▁ time ▁. ▁ You ▁ are ▁ incredible ▁, ▁ too ▁!“ ▁
Example 4: Sometimes ▁ they ▁ pretended ▁ it ▁ was ▁ a ▃ fire ▂ truck ▁ or ▄ a █ garbage ▅ truck ▄ or ▆ a ▇ delivery ▄ truck ▄. ▁
Click to see all of example 4
Ben ▁ and ▁ Lily ▁ liked ▁ to ▁ play ▁ with ▁ their ▁ toy ▁ truck ▁. ▁ It ▁ was ▁ big ▁ and ▁ red ▁ and ▁ had ▁ four ▁ wheels ▁. ▁ They ▁ could ▁ push ▁ it ▁ around ▁ the ▁ yard ▁ and ▁ make ▁ v ▁room ▁- ▁v ▁room ▁ noises ▁. ▁ Sometimes ▁ they ▁ pretended ▁ it ▁ was ▁ a ▃ fire ▂ truck ▁ or ▄ a █ garbage ▅ truck ▄ or ▆ a ▇ delivery ▄ truck ▄. ▁\n ▁\n ▁One ▁ day ▁, ▁ they ▁ decided ▁ to ▁ play ▁ delivery ▁ truck ▁. ▁ They ▁ looked ▁ for ▁ things ▁ to ▁ load ▁ in ▁ the ▁ back ▁ of ▁ the ▁ truck ▁. ▁ They ▁ found ▁ some ▁ rocks ▁, ▁ some ▁ leaves ▁, ▁ some ▁ sticks ▁ and ▁ some ▁ flowers ▁. ▁ They ▁ put ▁ them ▁ in ▁ the ▁ truck ▁ and ▁ said ▁, ▁ “ ▁This ▁ is ▁ our ▁ cargo ▁. ▁ We ▁ have ▁ to ▁ deliver ▁ it ▁ to ▁ our ▁ customers ▁.“ ▁\n ▁\n ▁They ▁ drove ▁ the ▁ truck ▁ around ▁ the ▁ yard ▁, ▁ stopping ▁ at ▁ different ▁ places ▁. ▁ They ▁ gave ▁ a ▁ rock ▁ to ▁ the ▂ dog ▁, ▂ a ▃ leaf ▃ to ▁ the ▃ bird ▁, ▃ a ▄ stick ▂ to ▁ the ▂ cat ▂ and ▂ a ▄ flower ▃ to ▁ the ▄ mom ▁. ▁ They ▁ said ▁, ▁ “ ▁Here ▁ is ▁ your ▁ cargo ▁. ▁ Thank ▁ you ▁ for ▁ choosing ▁ our ▁ delivery ▁ truck ▁.“ ▁\n ▁\n ▁Then ▁ they ▁ saw ▁ a ▁ bunch ▁ of ▁ bananas ▁ on ▁ the ▁ kitchen ▁ table ▁. ▁ They ▁ thought ▁, ▁ “ ▁Ban ▁anas ▁ are ▁ y ▁ummy ▁. ▁ We ▁ want ▁ some ▁ bananas ▁.“ ▁ They ▁ ran ▁ to ▁ the ▁ table ▁ and ▁ grabbed ▁ the ▁ bananas ▁. ▁ They ▁ put ▁ them ▁ in ▁ the ▁ truck ▁ and ▁ said ▁, ▁ “ ▁This ▁ is ▁ our ▁ special ▁ cargo ▁. ▁ We ▁ have ▁ to ▁ deliver ▁ it ▁ to ▁ ourselves ▁.“ ▁\n ▁\n ▁They ▁ drove ▁ the ▁ truck ▁ to ▁ their ▁ favorite ▁ spot ▁ under ▁ the ▁ tree ▁. ▁ They ▁ unloaded ▁ the ▁ bananas ▁ and ▁ peeled ▁ them ▁. ▁ They ▁ ate ▁ them ▁ and ▁ said ▁, ▁ “ ▁M ▁mm ▁, ▁ these ▁ are ▁ the ▁ best ▁ bananas ▁ ever ▁. ▁ We ▁ are ▁ mighty ▁ delivery ▁ truck ▁ drivers ▁. ▁ We ▁ can ▁ load ▁ and ▁ un ▁load ▁ anything ▁.“ ▁ They ▁ smiled ▁ and ▁ hugged ▁ each ▁ other ▁. ▁ They ▁ were ▁ happy ▁ and ▁ full ▁. ▁
Example 5: She ▁ pret ▁ends ▁ she ▁ is ▁ a ▂ mouse ▁ or ▃ a █ cat ▅ or ▅ a ▇ snake ▄. ▁
Click to see all of example 5
Anna ▁ likes ▁ to ▁ crawl ▁. ▁ She ▁ craw ▁ls ▁ under ▁ the ▁ table ▁, ▁ under ▁ the ▁ sofa ▁, ▂ under ▁ the ▁ bed ▁. ▁ She ▁ pret ▁ends ▁ she ▁ is ▁ a ▂ mouse ▁ or ▃ a █ cat ▅ or ▅ a ▇ snake ▄. ▁ She ▁ makes ▁ funny ▁ noises ▁ and ▁ gigg ▁les ▁. ▁\n ▁\n ▁One ▁ day ▁, ▁ she ▁ craw ▁ls ▁ under ▁ the ▁ window ▁ and ▁ sees ▁ a ▁ big ▁ aer ▁opl ▁ane ▁ in ▁ the ▁ sky ▁. ▁ It ▁ is ▁ white ▁ and ▁ shiny ▁ and ▁ has ▁ wings ▁ and ▁ a ▁ tail ▁. ▁ It ▁ makes ▁ a ▁ loud ▁ noise ▁ and ▁ flies ▁ very ▁ fast ▁. ▁ Anna ▁ is ▁ amazed ▁ and ▁ excited ▁. ▁ She ▁ wants ▁ to ▁ see ▁ more ▁ aer ▁opl ▁anes ▁. ▁\n ▁\n ▁She ▁ craw ▁ls ▁ out ▁ of ▁ the ▁ window ▁ and ▁ into ▁ the ▁ garden ▁. ▁ She ▁ looks ▁ up ▁ and ▁ sees ▁ many ▁ aer ▁opl ▁anes ▁. ▁ Some ▁ are ▁ big ▁, ▁ some ▁ are ▁ small ▁, ▁ some ▁ are ▁ red ▁, ▁ some ▁ are ▂ blue ▂. ▁ They ▁ fly ▁ in ▁ different ▁ directions ▁ and ▁ make ▁ different ▁ noises ▁. ▁ Anna ▁ thinks ▁ they ▁ are ▁ busy ▁. ▁ They ▁ must ▁ have ▁ many ▁ places ▁ to ▁ go ▁ and ▁ many ▁ things ▁ to ▁ do ▁. ▁\n ▁\n ▁She ▁ craw ▁ls ▁ to ▁ the ▁ fence ▁ and ▁ waves ▁ at ▁ the ▁ aer ▁opl ▁anes ▁. ▁ She ▁ hopes ▁ they ▁ see ▁ her ▁ and ▁ wave ▁ back ▁. ▁ She ▁ says ▁ hello ▁ and ▁ goodbye ▁ and ▁ thank ▁ you ▁ and ▁ please ▁. ▁ She ▁ thinks ▁ they ▁ are ▁ friendly ▁ and ▁ nice ▁. ▁\n ▁\n ▁She ▁ craw ▁ls ▁ back ▁ to ▁ the ▁ window ▁ and ▁ sees ▁ her ▁ mum ▁. ▁ Her ▁ mum ▁ smiles ▁ and ▁ picks ▁ her ▁ up ▁. ▁ She ▁ hugs ▁ her ▁ and ▁ kisses ▁ her ▁ and ▁ says ▁ she ▁ loves ▁ her ▁. ▁ She ▁ asks ▁ her ▁ what ▁ she ▁ was ▁ doing ▁. ▁ Anna ▁ tells ▁ her ▁ she ▁ was ▁ crawling ▁ and ▁ watching ▁ the ▁ aer ▁opl ▁anes ▁. ▁ She ▁ says ▁ they ▁ are ▁ busy ▁ and ▁ friendly ▁ and ▁ nice ▁. ▁ Her ▁ mum ▁ says ▁ she ▁ is ▁ clever ▁ and ▁ curious ▁ and ▁ brave ▁. ▁ She ▁ says ▁ they ▁ can ▁ watch ▁ the ▁ aer ▁opl ▁anes ▁ together ▁. ▁ Anna ▁ is ▁ happy ▁ and ▁ proud ▁. ▁ She ▁ loves ▁ her ▁ mum ▁ and ▁ the ▁ aer ▁opl ▁anes ▁. ▁
Example 6: They ▁ pretended ▁ they ▁ were ▁ crossing ▁ a ▁ jungle ▁ or ▅ a █ mountain ▅.
Click to see all of example 6
L ▁ily ▁ and ▁ Ben ▁ were ▁ best ▁ friends ▁. ▁ They ▁ liked ▁ to ▁ play ▁ in ▁ the ▁ park ▁ every ▁ day ▁. ▁ They ▁ had ▁ a ▁ lot ▁ of ▁ fun ▁ on ▁ the ▁ swings ▁, ▁ the ▁ slide ▁ and ▁ the ▃ sees ▁aw ▁. ▁ But ▁ their ▁ favorite ▁ thing ▁ was ▁ the ▁ rope ▁ bridge ▁. ▁ It ▁ was ▁ high ▁ and ▁ long ▁ and ▁ w ▁obb ▁ly ▁. ▁ They ▁ pretended ▁ they ▁ were ▁ crossing ▁ a ▁ jungle ▁ or ▅ a █ mountain ▅. ▁\n ▁\n ▁One ▁ day ▁, ▁ they ▁ saw ▁ a ▁ new ▁ boy ▁ in ▁ the ▁ park ▁. ▁ His ▁ name ▁ was ▁ Max ▁. ▁ He ▁ looked ▁ sad ▁ and ▁ lonely ▁. ▁ Lily ▁ and ▁ Ben ▁ wanted ▁ to ▁ be ▁ nice ▁ to ▁ him ▁. ▁ They ▁ asked ▁ him ▁ if ▁ he ▁ wanted ▁ to ▁ play ▁ with ▁ them ▁. ▁ Max ▁ nodded ▁ and ▁ smiled ▁. ▁ They ▁ showed ▁ him ▁ the ▁ rope ▁ bridge ▁ and ▁ told ▁ him ▁ it ▁ was ▁ fun ▁. ▁\n ▁\n ▁But ▁ Max ▁ was ▁ scared ▁ of ▁ the ▁ rope ▁ bridge ▁. ▁ He ▁ thought ▁ it ▁ was ▁ too ▁ high ▁ and ▁ too ▁ w ▁obb ▁ly ▁. ▁ He ▁ did ▁ not ▁ want ▁ to ▁ cross ▁ it ▁. ▁ He ▁ said ▁ he ▁ would ▁ wait ▁ for ▁ them ▁ on ▁ the ▁ other ▁ side ▁. ▁ Lily ▁ and ▁ Ben ▁ said ▁ okay ▁ and ▁ started ▁ to ▁ cross ▁ the ▁ rope ▁ bridge ▁. ▁ They ▁ held ▁ the ▁ rope ▁ tight ▁ and ▁ walked ▁ slowly ▁. ▁\n ▁\n ▁But ▁ when ▁ they ▁ were ▁ in ▁ the ▁ middle ▁ of ▁ the ▁ bridge ▁, ▁ they ▁ heard ▁ a ▁ loud ▁ snap ▁. ▁ One ▁ of ▁ the ▁ ropes ▁ had ▁ broken ▁. ▁ The ▁ bridge ▁ tilted ▁ and ▁ shook ▁. ▁ Lily ▁ and ▁ Ben ▁ screamed ▁. ▁ They ▁ were ▁ afraid ▁ they ▁ would ▁ fall ▁. ▁ They ▁ looked ▁ for ▁ Max ▁, ▁ but ▁ he ▁ was ▁ not ▁ there ▁. ▁ He ▁ had ▁ run ▁ away ▁. ▁\n ▁\n ▁L ▁ily ▁ and ▁ Ben ▁ did ▁ not ▁ know ▁ what ▁ to ▁ do ▁. ▁ They ▁ were ▁ stuck ▁ on ▁ the ▁ bridge ▁. ▁ They ▁ cried ▁ for ▁ help ▁. ▁ But ▁ no ▁ one ▁ heard ▁ them ▁. ▁ They ▁ wished ▁ Max ▁ had ▁ stayed ▁ with ▁ them ▁. ▁ They ▁ wished ▁ they ▁ had ▁ a ▁ friend ▁ who ▁ would ▁ not ▁ leave ▁ them ▁. ▁\n ▁\n ▁Then ▁ they ▁ saw ▁ a ▁ man ▁ coming ▁. ▁ He ▁ was ▁ wearing ▁ a ▁ hat ▁ and ▁ a ▁ badge ▁. ▁ He ▁ was ▁ a ▁ park ▁ ranger ▁. ▁ He ▁ saw ▁ Lily ▁ and ▁ Ben ▁ on ▁ the ▁ bridge ▁. ▁ He ▁ ran ▁ to ▁ help ▁ them ▁. ▁ He ▁ had ▁ a ▁ big ▁ rope ▁. ▁ He ▁ threw ▁ it ▁ to ▁ them ▁ and ▁ told ▁ them ▁ to ▁ hold ▁ it ▁ tight ▁. ▁ He ▁ pulled ▁ them ▁ to ▁ safety ▁. ▁ He ▁ hugged ▁ them ▁ and ▁ said ▁ they ▁ were ▁ brave ▁. ▁\n ▁\n ▁L ▁ily ▁ and ▁ Ben ▁ thanked ▁ the ▁ park ▁ ranger ▁. ▁ They ▁ were ▁ happy ▁ he ▁ had ▁ saved ▁ them ▁. ▁ They ▁ learned ▁ a ▁ lesson ▁ that ▁ day ▁. ▁ They ▁ learned ▁ that ▁ a ▁ true ▁ friend ▁ is ▁ someone ▁ who ▁ remains ▁ with ▁ you ▁ when ▁ you ▁ need ▁ them ▁. ▁ They ▁ learned ▁ that ▁ a ▁ rope ▁ can ▁ be ▁ tight ▁ or ▁ loose ▁, ▁ but ▁ a ▁ friendship ▁ should ▁ always ▁ be ▁ tight ▁. ▁
Example 7: Sometimes ▁ they ▁ pretended ▁ that ▁ the ▁ curtain ▁ was ▁ a ▂ door ▂ to ▁ a ▂ different ▁ place ▁, ▂ like ▁ a ▂ castle ▂ or ▅ a █ jungle ▅ or ▆ a ▇ spaceship ▇. ▁
Click to see all of example 7
L ▁ily ▁ and ▁ Ben ▁ were ▁ twins ▁ who ▁ liked ▁ to ▁ play ▁ in ▁ their ▁ room ▁. ▁ They ▁ had ▁ a ▁ big ▁ curtain ▁ that ▁ hung ▁ from ▁ the ▁ ceiling ▁ and ▁ made ▁ a ▁ wall ▁ in ▁ the ▁ middle ▁ of ▁ the ▁ room ▁. ▁ Sometimes ▁ they ▁ pretended ▁ that ▁ the ▁ curtain ▁ was ▁ a ▂ door ▂ to ▁ a ▂ different ▁ place ▁, ▂ like ▁ a ▂ castle ▂ or ▅ a █ jungle ▅ or ▆ a ▇ spaceship ▇. ▁\n ▁\n ▁One ▁ day ▁, ▁ they ▁ decided ▁ to ▁ play ▁ a ▁ game ▁ of ▁ hide ▁ and ▁ seek ▁. ▁ Lily ▁ said ▁, ▁ “ ▁I ▁ will ▁ count ▁ to ▁ ten ▁ and ▁ you ▁ hide ▁ behind ▁ the ▁ curtain ▁. ▁ Then ▁ I ▁ will ▁ try ▁ to ▁ find ▁ you ▁.“ ▁ Ben ▁ nodded ▁ and ▁ ran ▁ behind ▁ the ▁ curtain ▁. ▁ Lily ▁ closed ▁ her ▁ eyes ▁ and ▁ counted ▁ out ▁ loud ▁, ▁ “ ▁One ▁, ▃ two ▁, ▂ three ▁, ▁ four ▁, ▁ five ▁, ▁ six ▁, ▁ seven ▁, ▁ eight ▁, ▁ nine ▁, ▁ ten ▁! ▁ Ready ▁ or ▁ not ▁, ▁ here ▁ I ▁ come ▁!“ ▁\n ▁\n ▁L ▁ily ▁ opened ▁ her ▁ eyes ▁ and ▁ looked ▁ around ▁ the ▁ room ▁. ▁ She ▁ saw ▁ a ▁ small ▁ bump ▁ under ▁ the ▁ bed ▁, ▂ a ▂ pile ▁ of ▁ toys ▁ in ▃ the ▂ corner ▁, ▂ and ▂ a ▄ lamp ▁ on ▃ the ▁ table ▂. ▁ She ▁ thought ▁, ▁ “ ▁Where ▁ is ▁ Ben ▁? ▁ Maybe ▁ he ▁ is ▁ under ▁ the ▁ bed ▁.“ ▁ She ▁ walked ▁ to ▁ the ▁ bed ▁ and ▁ lifted ▁ the ▁ blanket ▁. ▁ But ▁ there ▁ was ▁ no ▁ Ben ▁, ▁ only ▁ a ▁ stuffed ▁ bear ▁. ▁ She ▁ said ▁, ▁ “ ▁O ▁ops ▁, ▁ not ▁ here ▁!“ ▁\n ▁\n ▁She ▁ moved ▁ to ▁ the ▁ corner ▁ and ▁ looked ▁ at ▁ the ▁ toys ▁. ▁ She ▁ saw ▁ a ▁ truck ▁, ▂ a ▄ ball ▂, ▄ a ▄ doll ▃, ▄ and ▄ a ▄ book ▂. ▁ She ▁ thought ▁, ▁ “ ▁Maybe ▁ he ▁ is ▁ behind ▁ the ▁ toys ▁.“ ▁ She ▁ pushed ▁ the ▁ toys ▁ aside ▁ and ▁ looked ▁ behind ▁ them ▁. ▁ But ▁ there ▁ was ▁ no ▁ Ben ▁, ▁ only ▁ a ▂ spider ▁ web ▁. ▁ She ▁ said ▁, ▁ “ ▁Y ▁uck ▁, ▁ not ▁ here ▁!“ ▁\n ▁\n ▁She ▁ walked ▁ to ▁ the ▁ table ▁ and ▁ looked ▁ at ▁ the ▁ lamp ▁. ▁ She ▁ saw ▁ a ▁ cord ▁, ▁ a ▃ switch ▁, ▃ a ▃ shade ▁, ▃ and ▃ a ▄ bulb ▁. ▁ She ▁ thought ▁, ▁ “ ▁Maybe ▁ he ▁ is ▁ under ▁ the ▁ lamp ▁.“ ▁ She ▁ lifted ▁ the ▁ lamp ▁ and ▁ looked ▁ under ▁ it ▁. ▁ But ▁ there ▁ was ▁ no ▁ Ben ▁, ▁ only ▂ a ▂ dust ▁ bunny ▁. ▁ She ▁ said ▁, ▁ “ ▁E ▁w ▁, ▁ not ▁ here ▁!“ ▁\n ▁\n ▁She ▁ scratched ▁ her ▁ head ▁ and ▁ wondered ▁, ▁ “ ▁Where ▁ is ▁ Ben ▁? ▁ He ▁ is ▁ very ▁ good ▁ at ▁ hiding ▁. ▁ Maybe ▁ he ▁ is ▁ behind ▁ the ▁ curtain ▁.“ ▁ She ▁ ran ▁ to ▁ the ▁ curtain ▁ and ▁ pulled ▁ it ▁ aside ▁. ▁ But ▁ there ▁ was ▁ no ▁ Ben ▁, ▁ only ▁ a ▂ big ▁ hole ▁. ▁ She ▁ gasped ▁ and ▁ said ▁, ▁ “ ▁Oh ▁ no ▁, ▁ the ▁ curtain ▁ is ▁ split ▁!“ ▁\n ▁\n ▁She ▁ heard ▁ a ▁ g ▁iggle ▁ from ▁ the ▁ other ▁ side ▁ of ▁ the ▁ hole ▁. ▁ She ▁ looked ▁ through ▁ it ▁ and ▁ saw ▁ Ben ▁. ▁ He ▁ was ▁ smiling ▁ and ▁ holding ▁ a ▁ pair ▁ of ▁ scissors ▁. ▁ He ▁ said ▁, ▁ “ ▁Sur ▁prise ▁! ▁ I ▁ made ▁ a ▁ new ▁ door ▁. ▁ Do ▁ you ▁ like ▁ it ▁?“ ▁ He ▁ was ▁ very ▁ playful ▁ and ▁ thought ▁ it ▁ was ▁ funny ▁. ▁\n ▁\n ▁L ▁ily ▁ was ▁ not ▁ amused ▁. ▁ She ▁ was ▁ angry ▁ and ▁ sad ▁. ▁ She ▁ said ▁, ▁ “ ▁Ben ▁, ▁ that ▁ was ▁ not ▁ nice ▁. ▁ You ▁ ruined ▁ the ▁ curtain ▁. ▁ Mom ▁ and ▁ Dad ▁ will ▁ be ▁ mad ▁. ▁ How ▁ will ▁ we ▁ fix ▁ it ▁?“ ▁ She ▁ started ▁ to ▁ cry ▁. ▁\n ▁\n ▁Ben ▁ realized ▁ that ▁ he ▁ had ▁ made ▁ a ▁ mistake ▁. ▁ He ▁ felt ▁ sorry ▁ and ▁ ashamed ▁. ▁ He ▁ said ▁, ▁ “ ▁I ▁’m ▁ sorry ▁, ▁ Lily ▁. ▁ I ▁ didn ▁’t ▁ mean ▁ to ▁ make ▁ you ▁ cry ▁. ▁ I ▁ just ▁ wanted ▁ to ▁ have ▁ fun ▁. ▁ Please ▁ don ▁’t ▁ be ▁ mad ▁ at ▁ me ▁. ▁ Maybe ▁ we ▁ can ▁ sew ▁ the ▁ curtain ▁ back ▁ together ▁. ▁ Or ▁ maybe ▁ we ▁ can ▁ find ▁ a ▁ new ▁ curtain ▁. ▁ Or ▁ maybe ▁ we ▁ can ▁ pretend ▁ that ▁ the ▁ hole ▁ is ▁ a ▁ window ▁. ▁ Can ▁ we ▁ still ▁ be ▁ friends ▁?“ ▁\n ▁\n ▁L ▁ily ▁ looked ▁ at ▁ Ben ▁ and ▁ saw ▁ that ▁ he ▁ was ▁ sincere ▁. ▁ She ▁ wiped ▁ her ▁ tears ▁ and ▁ said ▁, ▁ “ ▁OK ▁, ▁ Ben ▁. ▁ I ▁ forgive ▁ you ▁. ▁ But ▁ you ▁ have ▁ to ▁ promise ▁ not ▁ to ▁ cut ▁ the ▁ curtain ▁ again ▁. ▁ And ▁ you ▁ have ▁ to ▁ help ▁ me ▁ clean ▁ up ▁ the ▁ mess ▁. ▁ And ▁ you ▁ have ▁ to ▁ let ▁ me ▁ hide ▁ next ▁ time ▁. ▁ Deal ▁?“ ▁\n ▁\n ▁Ben ▁ nodded ▁ and ▁ said ▁, ▁ “ ▁Deal ▁. ▁ I ▁’m ▁ sorry ▁, ▁ Lily ▁. ▁ I ▁ love ▁ you ▁.“ ▁ He ▁ hugged ▁ her ▁ and ▁ said ▁, ▁ “ ▁You ▁ are ▁ the ▁ best ▁ sister ▁ ever ▁.“ ▁\n ▁\n ▁L ▁ily ▁ hugged ▁ him ▁ back ▁ and ▁ said ▁, ▁ “ ▁You ▁ are ▁ the ▁ best ▁ brother ▁ ever ▁. ▁ But ▁ you ▁ are ▁ also ▁ very ▁ naughty ▁. ▁ Come ▁ on ▁, ▁ let ▁’s ▁ go ▁ find ▁ Mom ▁ and ▁ Dad ▁ and ▁ tell ▁ them ▁ what ▁ happened ▁. ▁ Maybe ▁ they ▁ will ▁ not ▁ be ▁ too ▁ mad ▁ if ▁ we ▁ say ▁ we ▁ are ▁ sorry ▁ and ▁ we ▁ will ▁ fix ▁ it ▁.“ ▁\n ▁\n ▁They ▁ held ▁ hands ▁ and ▁ walked ▁ out ▁ of ▁ the ▁ room ▁. ▁ They ▁ hoped ▁ that ▁ Mom ▁ and ▁ Dad ▁ would ▁ understand ▁. ▁ They ▁ learned ▁ that ▁ playing ▁ is ▁ fun ▁, ▁ but ▁ not ▁ when ▁ it ▁ hurts ▁ someone ▁ or ▁ something ▁. ▁ They ▁ also ▁ learned ▁ that ▁ saying ▁ sorry ▁ and ▁ forgiving ▁ are ▁ important ▁ when ▁ you ▁ make ▁ a ▁ mistake ▁. ▁ They ▁ were ▁ still ▁ twins ▁ who ▁ liked ▁ to ▁ play ▁, ▁ but ▁ they ▁ were ▁ also ▁ more ▁ careful ▁ and ▁ kind ▁. ▁
Example 8: They ▁ can ▁ go ▁ inside ▁ and ▁ pretend ▁ it ▁ is ▁ a ▂ ship ▂ or ▄ a █ house ▆. ▁
Click to see all of example 8
L ▁ila ▁ and ▁ Tom ▁ are ▁ happy ▁. ▁ They ▁ like ▁ to ▁ play ▁ with ▁ their ▁ toys ▁ and ▁ books ▁. ▁ They ▁ have ▁ a ▁ big ▁ port ▁ in ▁ their ▁ room ▁. ▁ It ▁ is ▁ a ▁ big ▁ box ▁ with ▁ a ▁ hole ▁. ▁ They ▁ can ▁ go ▁ inside ▁ and ▁ pretend ▁ it ▁ is ▁ a ▂ ship ▂ or ▄ a █ house ▆. ▁\n ▁\n ▁One ▁ day ▁, ▁ L ▁ila ▁ has ▁ an ▁ idea ▁. ▁ She ▁ finds ▁ a ▁ big ▁ cloth ▁ in ▁ the ▁ closet ▁. ▁ She ▁ says ▁ to ▁ Tom ▁, ▁ “ ▁Let ▁’s ▁ wrap ▁ the ▁ port ▁ with ▁ this ▁ cloth ▁. ▁ It ▁ will ▁ be ▁ a ▁ secret ▁ port ▁. ▁ No ▁ one ▁ can ▁ see ▁ us ▁ inside ▁.“ ▁\n ▁\n ▁Tom ▁ likes ▁ the ▁ idea ▁. ▁ He ▁ helps ▁ L ▁ila ▁ wrap ▁ the ▁ port ▁ with ▁ the ▁ cloth ▁. ▁ They ▁ t ▁uck ▁ the ▁ ends ▁ under ▁ the ▁ box ▁. ▁ They ▁ make ▁ sure ▁ there ▁ is ▁ a ▁ gap ▁ for ▁ the ▁ hole ▁. ▁ They ▁ crawl ▁ inside ▁ the ▁ port ▁. ▁ It ▁ is ▁ dark ▁ and ▁ cozy ▁. ▁\n ▁\n ▁They ▁ g ▁iggle ▁ and ▁ whisper ▁. ▁ They ▁ tell ▁ each ▁ other ▁ stories ▁ and ▁ jokes ▁. ▁ They ▁ play ▁ with ▁ their ▁ toys ▁ and ▁ books ▁. ▁ They ▁ have ▁ fun ▁ in ▁ their ▁ secret ▁ port ▁. ▁\n ▁\n ▁Mom ▁ comes ▁ to ▁ check ▁ on ▁ them ▁. ▁ She ▁ sees ▁ the ▁ wrapped ▁ port ▁ in ▁ their ▁ room ▁. ▁ She ▁ smiles ▁. ▁ She ▁ knows ▁ they ▁ are ▁ inside ▁. ▁ She ▁ says ▁, ▁ “ ▁L ▁ila ▁, ▁ Tom ▁, ▁ are ▁ you ▁ in ▁ there ▁?“ ▁\n ▁\n ▁L ▁ila ▁ and ▁ Tom ▁ hear ▁ Mom ▁. ▁ They ▁ say ▁, ▁ “ ▁Yes ▁, ▁ Mom ▁, ▁ we ▁ are ▁ in ▁ the ▁ port ▁. ▁ It ▁ is ▁ our ▁ secret ▁ port ▁. ▁ Do ▁ you ▁ want ▁ to ▁ come ▁ in ▁?“ ▁\n ▁\n ▁Mom ▁ says ▁, ▁ “ ▁Sure ▁, ▁ I ▁ would ▁ love ▁ to ▁ come ▁ in ▁. ▁ Can ▁ I ▁ fit ▁ in ▁ the ▁ hole ▁?“ ▁\n ▁\n ▁L ▁ila ▁ and ▁ Tom ▁ say ▁, ▁ “ ▁Yes ▁, ▁ Mom ▁, ▁ you ▁ can ▁ fit ▁ in ▁ the ▁ hole ▁. ▁ Come ▁ in ▁, ▁ come ▁ in ▁.“ ▁\n ▁\n ▁Mom ▁ craw ▁ls ▁ in ▁ the ▁ hole ▁. ▁ She ▁ joins ▁ L ▁ila ▁ and ▁ Tom ▁ in ▁ the ▁ port ▁. ▁ They ▁ hug ▁ and ▁ kiss ▁. ▁ They ▁ are ▁ happy ▁. ▁ They ▁ have ▁ a ▁ secret ▁ port ▁. ▁
Example 9: They ▁ wondered ▁ if ▁ it ▁ was ▁ a ▁ lion ▁ or ▄ a █ bear ▃ or ▅ a ▇ monster ▃. ▁
Click to see all of example 9
L ▁ily ▁ and ▁ Ben ▁ were ▁ playing ▁ in ▁ the ▁ park ▁ with ▁ their ▁ toy ▁ cars ▁. ▁ They ▁ liked ▁ to ▁ make ▁ noises ▁ as ▁ they ▁ zoom ▁ed ▁ them ▁ around ▁ the ▁ grass ▁ and ▁ the ▂ sand ▁. ▁ Lily ▁ had ▁ a ▁ red ▁ car ▁ and ▁ Ben ▁ had ▁ a ▁ blue ▁ car ▁. ▁ They ▁ pretended ▁ they ▁ were ▁ racing ▁ each ▁ other ▁. ▁\n ▁\n ▁Suddenly ▁, ▁ they ▁ heard ▁ a ▁ loud ▁ roar ▁ from ▁ behind ▁ the ▁ trees ▁. ▁ They ▁ stopped ▁ playing ▁ and ▁ looked ▁ at ▁ each ▁ other ▁. ▁ They ▁ felt ▁ scared ▁. ▁ What ▁ could ▁ make ▁ such ▁ a ▁ big ▁ noise ▁? ▁ They ▁ wondered ▁ if ▁ it ▁ was ▁ a ▁ lion ▁ or ▄ a █ bear ▃ or ▅ a ▇ monster ▃. ▁\n ▁\n ▁They ▁ saw ▁ a ▁ flash ▁ of ▁ light ▁ from ▁ behind ▁ the ▁ trees ▁. ▁ It ▁ was ▁ bright ▁ and ▁ yellow ▁. ▁ They ▁ heard ▁ another ▁ roar ▁, ▂ louder ▁ than ▁ before ▁. ▁ They ▁ saw ▁ something ▁ big ▁ and ▁ brown ▁ come ▁ out ▁ of ▁ the ▁ trees ▁. ▁ It ▁ had ▁ a ▁ wide ▁ mouth ▁ and ▁ sharp ▁ teeth ▁. ▁ It ▁ was ▁ a ▁ crocod ▁ile ▁! ▁\n ▁\n ▁L ▁ily ▁ and ▁ Ben ▁ dropped ▁ their ▁ toy ▁ cars ▁ and ▁ ran ▁ away ▁ as ▁ fast ▁ as ▁ they ▁ could ▁. ▁ They ▁ screamed ▁ for ▁ help ▁. ▁ They ▁ saw ▁ their ▁ mom ▁ and ▁ dad ▁ sitting ▁ on ▁ a ▁ bench ▁ nearby ▁. ▁ They ▁ ran ▁ to ▁ them ▁ and ▁ hugged ▁ them ▁. ▁ They ▁ told ▁ them ▁ what ▁ they ▁ saw ▁. ▁\n ▁\n ▁Mom ▁ and ▁ dad ▁ looked ▁ at ▁ the ▁ trees ▁. ▁ They ▁ saw ▁ the ▁ light ▁ and ▁ the ▁ crocod ▁ile ▁ too ▁. ▁ But ▁ they ▁ also ▁ saw ▁ something ▁ else ▁. ▁ They ▁ saw ▁ a ▁ man ▁ with ▁ a ▁ camera ▁ and ▁ a ▂ speaker ▁. ▁ He ▁ was ▁ making ▁ the ▁ roar ▁ and ▁ the ▁ light ▁. ▁ He ▁ was ▁ making ▁ a ▁ movie ▁ about ▁ crocod ▁iles ▁. ▁\n ▁\n ▁Mom ▁ and ▁ dad ▁ laughed ▁ and ▁ explained ▁ to ▁ Lily ▁ and ▁ Ben ▁ that ▁ it ▁ was ▁ not ▁ a ▁ real ▁ crocod ▁ile ▁. ▁ It ▁ was ▁ a ▁ fake ▁ one ▁. ▁ It ▁ was ▁ just ▁ for ▁ fun ▁. ▁ They ▁ said ▁ they ▁ were ▁ sorry ▁ for ▁ sc ▁aring ▁ them ▁. ▁ They ▁ said ▁ they ▁ could ▁ go ▁ and ▁ see ▁ the ▁ movie ▁ when ▁ it ▁ was ▁ done ▁. ▁\n ▁\n ▁L ▁ily ▁ and ▁ Ben ▁ felt ▁ better ▁. ▁ They ▁ were ▁ not ▁ scared ▁ anymore ▁. ▁ They ▁ were ▁ curious ▁. ▁ They ▁ wanted ▁ to ▁ see ▁ the ▁ movie ▁ too ▁. ▁ They ▁ picked ▁ up ▁ their ▁ toy ▁ cars ▁ and ▁ went ▁ to ▁ the ▁ man ▁. ▁ They ▁ said ▁ hello ▁ and ▁ asked ▁ him ▁ questions ▁. ▁ He ▁ was ▁ nice ▁ and ▁ showed ▁ them ▁ how ▁ he ▁ made ▁ the ▁ roar ▁ and ▁ the ▁ light ▁. ▁ He ▁ let ▁ them ▁ touch ▁ the ▁ fake ▁ crocod ▁ile ▁. ▁ It ▁ was ▁ soft ▁ and ▁ smooth ▁. ▁\n ▁\n ▁L ▁ily ▁ and ▁ Ben ▁ had ▁ fun ▁. ▁ They ▁ learned ▁ something ▁ new ▁. ▁ They ▁ liked ▁ the ▁ movie ▁ man ▁ and ▁ the ▁ fake ▁ crocod ▁ile ▁. ▁ They ▁ said ▁ thank ▁ you ▁ and ▁ goodbye ▁. ▁ They ▁ went ▁ back ▁ to ▁ playing ▁ with ▁ their ▁ toy ▁ cars ▁. ▁ They ▁ still ▁ made ▁ noises ▁, ▁ but ▁ they ▁ also ▁ made ▁ ro ▁ars ▁ and ▁ lights ▁. ▁ They ▁ pretended ▁ they ▁ were ▁ crocod ▁iles ▁ too ▁. ▁
Example 10: Something ▁ that ▁ likes ▁ to ▁ fly ▁. ▁ Like ▁ a ▂ bird ▂ or ▁ a ▆ plane ▄ or ▄ a █ k ▅ite ▄,\" ▁ Lily ▁ said ▁
Click to see all of example 10
L ▁ily ▁ and ▁ Ben ▁ were ▁ playing ▁ with ▁ their ▁ toys ▁ in ▁ the ▁ backyard ▁. ▁ They ▁ had ▁ a ▁ lot ▁ of ▁ fun ▁ cars ▁, ▁ trucks ▁, ▁ and ▁ trains ▁ to ▁ make ▁ noises ▁ and ▁ zoom ▁ around ▁. ▁ But ▁ Lily ▁’s ▁ favorite ▁ toy ▁ was ▁ her ▁ crane ▁. ▁ It ▁ was ▁ big ▁ and ▁ yellow ▁ and ▁ had ▁ a ▁ long ▁ arm ▁ that ▁ could ▁ lift ▁ things ▁ up ▁ and ▁ down ▁. ▁\n ▁\n ▁“ ▁Look ▁, ▁ Ben ▁, ▁ I ▁ can ▁ put ▁ this ▁ ball ▁ on ▁ the ▁ crane ▁ and ▁ make ▁ it ▁ fly ▁!“ ▁ Lily ▁ said ▁, ▁ as ▁ she ▁ hooked ▁ the ▁ ball ▁ to ▁ the ▁ crane ▁’s ▁ arm ▁ and ▁ pressed ▁ a ▁ button ▁. ▁ The ▁ crane ▁ lifted ▁ the ▁ ball ▁ high ▁ in ▁ the ▁ air ▁ and ▁ then ▁ lowered ▁ it ▁ down ▁ again ▁. ▁\n ▁\n ▁“ ▁Wow ▁, ▁ that ▁’s ▁ cool ▁, ▁ Lily ▁! ▁ Can ▁ I ▁ try ▁?“ ▁ Ben ▁ asked ▁, ▁ reaching ▁ for ▁ the ▁ crane ▁. ▁\n ▁\n ▁“ ▁OK ▁, ▁ but ▁ be ▁ careful ▁. ▁ It ▁’s ▁ my ▁ crane ▁ and ▁ I ▁ love ▁ it ▁ very ▁ much ▁,“ ▁ Lily ▁ said ▁, ▁ handing ▁ the ▁ crane ▁ to ▁ Ben ▁. ▁\n ▁\n ▁Ben ▁ smiled ▁ and ▁ took ▁ the ▁ crane ▁. ▁ He ▁ looked ▁ for ▁ something ▁ to ▁ put ▁ on ▁ the ▁ crane ▁. ▁ He ▁ saw ▁ a ▁ small ▁, ▁ fluffy ▁, ▁ white ▁ cat ▁ sleeping ▁ on ▁ a ▁ chair ▁. ▁ The ▁ cat ▁ was ▁ adorable ▁ and ▁ looked ▁ very ▁ soft ▁. ▁\n ▁\n ▁“ ▁I ▁ know ▁, ▁ I ▁’ll ▁ put ▁ the ▁ cat ▁ on ▁ the ▁ crane ▁ and ▁ make ▁ it ▁ fly ▁ too ▁!“ ▁ Ben ▁ said ▁, ▁ as ▁ he ▁ grabbed ▁ the ▁ cat ▁ and ▁ tried ▁ to ▁ hook ▁ it ▁ to ▁ the ▁ crane ▁’s ▁ arm ▁. ▁\n ▁\n ▁But ▁ the ▁ cat ▁ did ▁ not ▁ like ▁ that ▁ at ▁ all ▁. ▁ It ▁ his ▁sed ▁ and ▁ scratched ▁ and ▁ bit ▁ Ben ▁’s ▁ hand ▁. ▁ Ben ▁ dropped ▁ the ▁ cat ▁ and ▁ the ▁ crane ▁ and ▁ cried ▁ out ▁ loud ▁. ▁\n ▁\n ▁“ ▁O ▁w ▁, ▁ ow ▁, ▁ ow ▁! ▁ The ▁ cat ▁ hurt ▁ me ▁!“ ▁ Ben ▁ said ▁, ▁ holding ▁ his ▁ hand ▁. ▁\n ▁\n ▁L ▁ily ▁ ran ▁ to ▁ Ben ▁ and ▁ saw ▁ his ▁ hand ▁ bleeding ▁. ▁ She ▁ felt ▁ sorry ▁ for ▁ him ▁ and ▁ hugged ▁ him ▁. ▁\n ▁\n ▁“ ▁I ▁’m ▁ sorry ▁, ▁ Ben ▁. ▁ The ▁ cat ▁ didn ▁’t ▁ want ▁ to ▁ fly ▁. ▁ It ▁ was ▁ scared ▁ and ▁ angry ▁. ▁ You ▁ should ▁ not ▁ put ▁ the ▁ cat ▁ on ▁ the ▁ crane ▁. ▁ That ▁’s ▁ not ▁ nice ▁,“ ▁ Lily ▁ said ▁. ▁\n ▁\n ▁“ ▁I ▁’m ▁ sorry ▁, ▁ Lily ▁. ▁ I ▁ didn ▁’t ▁ know ▁ the ▁ cat ▁ would ▁ do ▁ that ▁. ▁ I ▁ just ▁ wanted ▁ to ▁ have ▁ fun ▁. ▁ I ▁’m ▁ sorry ▁ I ▁ broke ▁ your ▁ crane ▁ too ▁,“ ▁ Ben ▁ said ▁. ▁\n ▁\n ▁L ▁ily ▁ looked ▁ at ▁ her ▁ crane ▁ and ▁ saw ▁ that ▁ it ▁ was ▁ not ▁ broken ▁. ▁ It ▁ was ▁ just ▁ a ▁ little ▂ dirty ▁. ▁ She ▁ picked ▁ it ▁ up ▁ and ▁ wiped ▁ it ▁ with ▁ a ▁ cloth ▁. ▁\n ▁\n ▁“ ▁It ▁’s ▁ OK ▁, ▁ Ben ▁. ▁ My ▁ crane ▁ is ▁ fine ▁. ▁ It ▁’s ▁ still ▁ big ▁ and ▁ yellow ▁ and ▁ can ▁ lift ▁ things ▁ up ▁ and ▁ down ▁. ▁ But ▁ next ▁ time ▁, ▁ let ▁’s ▁ put ▁ something ▁ else ▁ on ▁ the ▁ crane ▁. ▁ Something ▁ that ▁ likes ▁ to ▁ fly ▁. ▁ Like ▁ a ▂ bird ▂ or ▁ a ▆ plane ▄ or ▄ a █ k ▅ite ▄,“ ▁ Lily ▁ said ▁. ▁\n ▁\n ▁“ ▁OK ▁, ▁ Lily ▁. ▁ That ▁ sounds ▁ like ▁ a ▁ good ▁ idea ▁. ▁ I ▁’m ▁ sorry ▁ I ▁ made ▁ you ▁ and ▁ the ▁ cat ▁ sad ▁. ▁ Can ▁ we ▁ still ▁ be ▁ friends ▁?“ ▁ Ben ▁ asked ▁. ▁\n ▁\n ▁“ ▁Of ▁ course ▁, ▁ Ben ▁. ▁ We ▁ are ▁ always ▁ friends ▁. ▁ And ▁ the ▁ cat ▁ is ▁ OK ▁ too ▁. ▁ Look ▁, ▁ it ▁’s ▁ pur ▁ring ▁ on ▁ the ▁ chair ▁ again ▁. ▁ It ▁’s ▁ adorable ▁,“ ▁ Lily ▁ said ▁. ▁\n ▁\n ▁They ▁ smiled ▁ and ▁ hugged ▁ and ▁ went ▁ back ▁ to ▁ playing ▁ with ▁ their ▁ toys ▁. ▁ They ▁ had ▁ a ▁ lot ▁ of ▁ fun ▁ with ▁ the ▁ crane ▁ and ▁ other ▁ things ▁ that ▁ liked ▁ to ▁ fly ▁. ▁ And ▁ they ▁ never ▁ put ▁ the ▁ cat ▁ on ▁ the ▁ crane ▁ again ▁. ▁
Feature 2
My interpretation: This feature activates on “time” in “it was time to/for”, usually in a phrase like “the characters were having fun, but then it was time for something less fun”.
GPT-4o’s interpretation: “‘time’ is always strongly highlighted.”
Example 1: It ▁ was ▁ so ▁ much ▁ fun ▁! ▁ ▁\n ▁\n ▁But ▁ then ▁ it ▁ was ▁ time █ to ▁ go ▁ home ▁. ▁
Click to see all of example 1
Today ▁ was ▁ ordinary ▁. ▁ Emma ▁â ▁€ ▁™ ▁s ▁ mom ▁ took ▁ her ▁ to ▁ the ▁ park ▁. ▁ They ▁ played ▁ tag ▁, ▁ and ▁ swung ▁ on ▁ the ▁ swings ▁. ▁ It ▁ was ▁ so ▁ much ▁ fun ▁! ▁ ▁\n ▁\n ▁But ▁ then ▁ it ▁ was ▁ time █ to ▁ go ▁ home ▁. ▁ Emma ▁ felt ▁ sad ▁. ▁ She ▁ hugged ▁ her ▁ mom ▁ and ▁ said ▁, ▁ � ▁� ▁€ ▁� ▁� ▁I ▁ miss ▁ the ▁ park ▁. ▁â ▁€ ▁ ▁\n ▁\n ▁Her ▁ mom ▁ smiled ▁ and ▁ said ▁, ▁ � ▁� ▁€ ▁� ▁� ▁Me ▁ too ▁. ▁ But ▁ it ▁ will ▁ still ▁ be ▁ here ▁ tomorrow ▁. ▁â ▁€ ▁ ▁\n ▁\n ▁So ▁, ▁ Emma ▁ said ▁ goodbye ▁ and ▁ went ▁ inside ▁. ▁ She ▁ wished ▁ she ▁ could ▁ stay ▁ and ▁ play ▁ forever ▁, ▁ but ▁ tomorrow ▁ would ▁ come ▁. ▁ And ▁ she ▁ knew ▁ she ▁ would ▁ miss ▁ the ▁ park ▁ today ▁, ▁ but ▁ she ▁ could ▁ come ▁ back ▁ tomorrow ▁ to ▁ play ▁ again ▁. ▁
Example 2: They ▁ had ▁ so ▁ much ▁ fun ▁ pretending ▁ to ▁ be ▁ a ▁ plane ▁, ▁ but ▁ it ▁ was ▁ time █ for ▁ them ▁ to ▁ go ▁ home ▁. ▁
Click to see all of example 2
Once ▁ upon ▁ a ▁ time ▁, ▁ there ▁ were ▁ two ▁ friends ▁, ▁ Jack ▁ and ▁ Jill ▁. ▁ They ▁ were ▁ both ▁ so ▁ excited ▁ to ▁ play ▁ together ▁ outdoors ▁. ▁ They ▁ looked ▁ up ▁ and ▁ saw ▁ a ▁ beautiful ▁ white ▁ bird ▁ soaring ▁ in ▁ the ▁ sky ▁. ▁\n ▁\n ▁â ▁€ ▁� ▁� ▁Wow ▁, ▁ look ▁ at ▁ that ▁ bird ▁ soar ▁! ▁â ▁€ ▁ shouted ▁ Jack ▁. ▁ ▁\n ▁\n ▁J ▁ill ▁ replied ▁, ▁ � ▁� ▁€ ▁� ▁� ▁Let ▁â ▁€ ▁™ ▁s ▁ be ▁ like ▁ the ▁ bird ▁ and ▁ soar ▁ too ▁! ▁â ▁€ ▁\n ▁\n ▁Jack ▁ saw ▁ a ▁ broken ▁ branch ▁ on ▁ the ▁ ground ▁, ▁ so ▁ he ▁ picked ▁ it ▁ up ▁ and ▁ said ▁, ▁ � ▁� ▁€ ▁� ▁� ▁Let ▁â ▁€ ▁™ ▁s ▁ pretend ▁ this ▁ branch ▁ is ▁ like ▁ a ▁ plane ▁. ▁â ▁€ ▁\n ▁\n ▁So ▁ Jack ▁ and ▁ Jill ▁ hugged ▁ the ▁ branch ▁, ▁ closed ▁ their ▁ eyes ▁ and ▁ imagined ▁ they ▁ were ▁ soaring ▁ in ▁ the ▁ sky ▁. ▁ They ▁ hum ▁med ▁ and ▁ soared ▁ around ▁ each ▁ other ▁, ▁ pretending ▁ to ▁ make ▁ plane ▁ noises ▁. ▁\n ▁\n ▁They ▁ had ▁ so ▁ much ▁ fun ▁ pretending ▁ to ▁ be ▁ a ▁ plane ▁, ▁ but ▁ it ▁ was ▁ time █ for ▁ them ▁ to ▁ go ▁ home ▁. ▁ They ▁ hugged ▁ the ▁ branch ▁ one ▁ last ▁ time ▁, ▁ thanked ▁ it ▁ for ▁ the ▁ fun ▁, ▁ and ▁ said ▁ goodbye ▁. ▁
Example 3: He ▁ felt ▁ so ▁ happy ▁ for ▁ his ▁ new ▁ adventure ▁. ▁\n ▁\n ▁The ▁ sun ▁ was ▁ setting ▁ and ▁ it ▁ was ▁ time █ for ▁ Ted ▁ to ▁ go ▁ home ▁, ▁
Click to see all of example 3
Once ▁ there ▁ was ▁ a ▁ nice ▁ little ▁ boy ▁ named ▁ Ted ▁. ▁ He ▁ liked ▁ to ▁ climb ▁ lots ▁ of ▁ things ▁ like ▁ fences ▁, ▁ trees ▁ and ▁ rocks ▁. ▁ Today ▁ Ted ▁ woke ▁ up ▁ feeling ▁ extra ▁ special ▁. ▁ He ▁ decided ▁ to ▁ explore ▁ a ▁ new ▁ place ▁ and ▁ try ▁ something ▁ he ▁ had ▁ never ▁ done ▁ before ▁. ▁\n ▁\n ▁Ted ▁ thought ▁ that ▁ climbing ▁ a ▁ mountain ▁ would ▁ be ▁ exciting ▁. ▁ So ▁ he ▁ asked ▁ his ▁ mom ▁, ▁ � ▁� ▁€ ▁� ▁� ▁Mom ▁ can ▁ I ▁ go ▁ mountain ▁ climbing ▁? ▁â ▁€ ▁\n ▁\n ▁â ▁€ ▁� ▁� ▁Oh ▁ my ▁, ▁â ▁€ ▁ said ▁ his ▁ mom ▁, ▁ � ▁� ▁€ ▁� ▁� ▁that ▁’s ▁ a ▁ little ▁ too ▁ difficult ▁ for ▁ you ▁ right ▁ now ▁ Ted ▁, ▁ but ▁ I ▁ know ▁ a ▁ place ▁ you ▁ can ▁ go ▁. ▁ It ▁ has ▁ a ▁ kind ▁ of ▁ flame ▁ at ▁ the ▁ top ▁. ▁ It ▁’s ▁ called ▁ a ▁ lighthouse ▁. ▁â ▁€ ▁\n ▁\n ▁Ted ▁’s ▁ face ▁ lit ▁ up ▁ with ▁ excitement ▁. ▁ He ▁ knew ▁ his ▁ mom ▁ was ▁ right ▁, ▁ climbing ▁ a ▁ lighthouse ▁ would ▁ be ▁ much ▁ better ▁ than ▁ climbing ▁ a ▁ mountain ▁. ▁\n ▁\n ▁Ted ▁ put ▁ his ▁ best ▁ shoes ▁ and ▁ grabbed ▁ his ▁ hat ▁. ▁ He ▁ said ▁ goodbye ▁ to ▁ his ▁ mom ▁ and ▁ started ▁ running ▁ towards ▁ the ▁ lighthouse ▁. ▁\n ▁\n ▁As ▁ Ted ▁ was ▁ getting ▁ closer ▁ he ▁ could ▁ see ▁ the ▁ huge ▁ tower ▁ and ▁ the ▁ kind ▁ flame ▁ at ▁ the ▁ top ▁. ▁ He ▁ stopped ▁ to ▁ take ▁ a ▁ breath ▁ and ▁ then ▁ climbed ▁ the ▁ stairs ▁ until ▁ he ▁ reached ▁ the ▁ top ▁. ▁\n ▁\n ▁Ted ▁ looked ▁ around ▁, ▁ he ▁ could ▁ see ▁ for ▁ miles ▁ in ▁ every ▁ direction ▁. ▁ He ▁ felt ▁ so ▁ happy ▁ for ▁ his ▁ new ▁ adventure ▁. ▁\n ▁\n ▁The ▁ sun ▁ was ▁ setting ▁ and ▁ it ▁ was ▁ time █ for ▁ Ted ▁ to ▁ go ▁ home ▁, ▁ but ▁ he ▁ knew ▁ he ▁ would ▁ be ▁ back ▁ to ▁ climb ▁ his ▁ lighthouse ▁ again ▁ soon ▁. ▁
Example 4: Tom ▁ and ▁ Sue ▁ laughed ▁ and ▁ had ▁ a ▁ lot ▁ of ▁ fun ▁ together ▁. ▁ ▁\n ▁\n ▁Finally ▁, ▁ it ▁ was ▁ time █ for ▁ Tom ▁ to ▁ go ▁ home ▁. ▁
Click to see all of example 4
Once ▁ upon ▁ a ▁ time ▁, ▁ there ▁ was ▁ a ▁ deaf ▁ boy ▁ called ▁ Tom ▁. ▁ Tom ▁ liked ▁ to ▁ go ▁ for ▁ walks ▁. ▁ One ▁ day ▁, ▁ Tom ▁ asked ▁ his ▁ Mom ▁my ▁ if ▁ he ▁ could ▁ go ▁ for ▁ a ▁ visit ▁. ▁ His ▁ Mom ▁my ▁ said ▁ � ▁� ▁€ ▁� ▁� ▁Yes ▁, ▁ you ▁ can ▁ go ▁ for ▁ a ▁ visit ▁. ▁ But ▁ make ▁ sure ▁ you ▁â ▁€ ▁™ ▁re ▁ home ▁ before ▁ dinner ▁. ▁â ▁€ ▁ So ▁ Tom ▁ put ▁ on ▁ his ▁ shoes ▁ to ▁ go ▁ for ▁ a ▁ walk ▁. ▁\n ▁\n ▁On ▁ his ▁ walk ▁, ▁ Tom ▁ met ▁ a ▁ deaf ▁ girl ▁ called ▁ Sue ▁. ▁ Sue ▁ said ▁ to ▁ Tom ▁, ▁ � ▁� ▁€ ▁� ▁� ▁Hi ▁! ▁ What ▁ are ▁ you ▁ doing ▁? ▁â ▁€ ▁ Tom ▁ smiled ▁ and ▁ said ▁, ▁ � ▁� ▁€ ▁� ▁� ▁I ▁â ▁€ ▁™ ▁m ▁ going ▁ for ▁ a ▁ visit ▁. ▁ Would ▁ you ▁ like ▁ to ▁ come ▁ too ▁? ▁â ▁€ ▁ Sue ▁ nodded ▁, ▁ so ▁ Tom ▁ and ▁ Sue ▁ went ▁ for ▁ a ▁ walk ▁ together ▁. ▁ ▁\n ▁\n ▁They ▁ went ▁ to ▁ a ▁ park ▁ and ▁ looked ▁ at ▁ the ▁ birds ▁. ▁ They ▁ watched ▁ the ▁ squirrel ▁s ▁ and ▁ played ▁ on ▁ the ▁ swings ▁. ▁ Tom ▁ and ▁ Sue ▁ laughed ▁ and ▁ had ▁ a ▁ lot ▁ of ▁ fun ▁ together ▁. ▁ ▁\n ▁\n ▁Finally ▁, ▁ it ▁ was ▁ time █ for ▁ Tom ▁ to ▁ go ▁ home ▁. ▁ He ▁ said ▁ goodbye ▁ to ▁ Sue ▁ and ▁ thanked ▁ her ▁ for ▁ coming ▁ with ▁ him ▁ on ▁ his ▁ visit ▁. ▁ Then ▁ Tom ▁ waved ▁ goodbye ▁, ▁ and ▁ went ▁ for ▁ a ▁ walk ▁ back ▁ home ▁. ▁
Example 5: it ▁ was ▁ so ▁ much ▁ fun ▁. ▁ ▁\n ▁\n ▁But ▁ then ▁ one ▁ day ▁ Johnny ▁'s ▁ mom ▁ said ▁ it ▁ was ▁ time █ for ▁ bed ▁. ▁
Click to see all of example 5
Once ▁ upon ▁ a ▁ time ▁ there ▁ was ▁ a ▁ boy ▁ called ▁ Johnny ▁. ▁ He ▁ was ▁ very ▁ excited ▁ one ▁ day ▁ because ▁ he ▁ was ▁ going ▁ to ▁ learn ▁ a ▁ new ▁ thing ▁ � ▁� ▁€ ▁� ▁� ▁ how ▁ to ▁ sign ▁. ▁ His ▁ teacher ▁ taught ▁ him ▁ how ▁ to ▁ make ▁ lots ▁ of ▁ different ▁ signs ▁ with ▁ his ▁ hands ▁ and ▁ they ▁ were ▁ very ▁ fun ▁. ▁ ▁\n ▁\n ▁Next ▁, ▁ his ▁ mom ▁ gave ▁ him ▁ a ▁ ball ▁ of ▁ wool ▁. ▁ It ▁ was ▁ soft ▁ and ▁ fluffy ▁ and ▁ he ▁ really ▁ liked ▁ it ▁. ▁ He ▁ played ▁ with ▁ it ▁ every ▁ day ▁. ▁ He ▁ made ▁ lots ▁ of ▁ shapes ▁ and ▁ patterns ▁ with ▁ it ▁, ▁ like ▁ a ▁ hat ▁ and ▁ a ▁ scarf ▁. ▁ His ▁ mom ▁ cl ▁apped ▁ and ▁ smiled ▁, ▁ it ▁ was ▁ so ▁ much ▁ fun ▁. ▁ ▁\n ▁\n ▁But ▁ then ▁ one ▁ day ▁ Johnny ▁’s ▁ mom ▁ said ▁ it ▁ was ▁ time █ for ▁ bed ▁. ▁ But ▁ bed ▁time ▁ was ▁ so ▁ boring ▁ � ▁� ▁€ ▁� ▁� ▁ Johnny ▁ wanted ▁ to ▁ keep ▁ playing ▁ with ▁ the ▁ wool ▁ instead ▁! ▁ He ▁ tried ▁ to ▁ sneak ▁ it ▁ in ▁ his ▁ bedroom ▁ but ▁ his ▁ mom ▁ caught ▁ him ▁ and ▁ said ▁ he ▁ had ▁ to ▁ go ▁ to ▁ sleep ▁. ▁ ▁\n ▁\n ▁Johnny ▁ sighed ▁, ▁ but ▁ then ▁ he ▁ thought ▁ of ▁ something ▁. ▁ He ▁ made ▁ a ▁ sign ▁ with ▁ his ▁ hands ▁ and ▁ his ▁ mom ▁ smiled ▁ � ▁� ▁€ ▁� ▁� ▁ it ▁ was ▁ his ▁ own ▁ sign ▁ for ▁ � ▁� ▁€ ▁� ▁� ▁w ▁ool ▁â ▁€ ▁. ▁ His ▁ mom ▁ gave ▁ him ▁ the ▁ wool ▁ and ▁ he ▁ sn ▁ugg ▁led ▁ with ▁ it ▁ all ▁ the ▁ way ▁ to ▁ bed ▁. ▁\n ▁\n ▁The ▁ End ▁. ▁
Example 6: They ▁ waited ▁ for ▁ a ▁ long ▁ time ▁, ▁ but ▁ the ▁ raven ▁ never ▁ came ▁ back ▁. ▁ Eventually ▁, ▁ it ▁ was ▁ time █ to ▁ go ▁ home ▁
Click to see all of example 6
James ▁ and ▁ his ▁ mom ▁ were ▁ walking ▁ to ▁ the ▁ park ▁ one ▁ day ▁. ▁ James ▁ saw ▁ a ▁ beautiful ▁ raven ▁ flying ▁ in ▁ the ▁ sky ▁. ▁ He ▁ asked ▁ his ▁ mom ▁, ▁ � ▁� ▁€ ▁˜ ▁what ▁ is ▁ that ▁? ▁â ▁€ ▁™ ▁ She ▁ said ▁ it ▁ was ▁ a ▁ raven ▁. ▁ He ▁ asked ▁ what ▁ a ▁ raven ▁ was ▁. ▁ Mom ▁ said ▁, ▁ � ▁� ▁€ ▁� ▁� ▁A ▁ raven ▁ is ▁ a ▁ big ▁, ▁ black ▁ bird ▁. ▁â ▁€ ▁\n ▁\n ▁James ▁ wanted ▁ to ▁ see ▁ the ▁ raven ▁, ▁ so ▁ his ▁ mom ▁ and ▁ him ▁ ran ▁ to ▁ the ▁ park ▁. ▁ But ▁, ▁ when ▁ they ▁ arrived ▁, ▁ the ▁ raven ▁ was ▁ gone ▁. ▁ James ▁ asked ▁ where ▁ the ▁ raven ▁ went ▁ and ▁ his ▁ mom ▁ said ▁, ▁ � ▁� ▁€ ▁� ▁� ▁It ▁ was ▁ an ▁ unknown ▁ bird ▁, ▁ so ▁ we ▁ won ▁â ▁€ ▁™ ▁t ▁ know ▁ where ▁ it ▁ went ▁. ▁â ▁€ ▁\n ▁\n ▁James ▁ and ▁ his ▁ mom ▁ sat ▁ in ▁ the ▁ park ▁, ▁ looking ▁ for ▁ the ▁ raven ▁. ▁ They ▁ waited ▁ for ▁ a ▁ long ▁ time ▁, ▁ but ▁ the ▁ raven ▁ never ▁ came ▁ back ▁. ▁ Eventually ▁, ▁ it ▁ was ▁ time █ to ▁ go ▁ home ▁ and ▁ the ▁ raven ▁ had ▁ not ▁ returned ▁. ▁ James ▁ asked ▁ what ▁ happened ▁ to ▁ the ▁ bird ▁ and ▁ his ▁ mom ▁ answered ▁, ▁ � ▁� ▁€ ▁� ▁� ▁We ▁ may ▁ never ▁ know ▁. ▁ This ▁ is ▁ the ▁ end ▁ of ▁ the ▁ raven ▁. ▁â ▁€ ▁ ▁\n ▁\n ▁James ▁ was ▁ sad ▁ because ▁ his ▁ mom ▁ said ▁ the ▁ raven ▁ was ▁ gone ▁. ▁ He ▁ told ▁ his ▁ mom ▁, ▁ � ▁� ▁€ ▁� ▁� ▁I ▁ wish ▁ I ▁ could ▁ have ▁ found ▁ the ▁ raven ▁. ▁â ▁€ ▁ His ▁ mom ▁ replied ▁, ▁ “ ▁Me ▁ too ▁, ▁ James ▁. ▁ Me ▁ too ▁.“ ▁
Example 7: So ▁ the ▁ family ▁ settled ▁ at ▁ the ▁ playground ▁ and ▁ had ▁ a ▁ wonderful ▁ time ▁ playing ▁ and ▁ exploring ▁. ▁ When ▁ it ▁ was ▁ time █ to ▁ go ▁, ▁
Click to see all of example 7
Once ▁ upon ▁ a ▁ time ▁ there ▁ was ▁ a ▁ family ▁ that ▁ was ▁ very ▁ careful ▁. ▁ On ▁ an ▁ especially ▁ careful ▁ day ▁, ▁ they ▁ decided ▁ to ▁ go ▁ on ▁ an ▁ adventure ▁. ▁ The ▁ family ▁ loaded ▁ up ▁ their ▁ luggage ▁ into ▁ the ▁ car ▁ and ▁ everyone ▁ settled ▁ in ▁ for ▁ the ▁ ride ▁. ▁ ▁\n ▁\n ▁When ▁ they ▁ reached ▁ their ▁ destination ▁, ▁ the ▁ family ▁ unloaded ▁ their ▁ luggage ▁ and ▁ set ▁ off ▁ to ▁ explore ▁. ▁ As ▁ they ▁ were ▁ walking ▁, ▁ the ▁ 3 ▁ year ▁ old ▁ noticed ▁ something ▁ in ▁ the ▁ distance ▁. ▁ ▁\n ▁\n ▁The ▁ 3 ▁ year ▁ old ▁ excited ▁ly ▁ said ▁ to ▁ the ▁ parents ▁, ▁ “ ▁What ▁ is ▁ that ▁? ▁â ▁€ ▁\n ▁\n ▁The ▁ parents ▁ replied ▁, ▁ � ▁� ▁€ ▁� ▁� ▁That ▁ looks ▁ like ▁ a ▁ playground ▁! ▁ Let ▁’s ▁ go ▁ check ▁ it ▁ out ▁! ▁ Be ▁ careful ▁ though ▁ and ▁ make ▁ sure ▁ your ▁ luggage ▁ is ▁ secure ▁. ▁â ▁€ ▁\n ▁\n ▁So ▁ the ▁ family ▁ settled ▁ at ▁ the ▁ playground ▁ and ▁ had ▁ a ▁ wonderful ▁ time ▁ playing ▁ and ▁ exploring ▁. ▁ When ▁ it ▁ was ▁ time █ to ▁ go ▁, ▁ the ▁ family ▁ carefully ▁ loaded ▁ their ▁ luggage ▁ back ▁ in ▁ the ▁ car ▁ and ▁ went ▁ on ▁ their ▁ way ▁. ▁ ▁\n ▁\n ▁The ▁ end ▁. ▁
Example 8: Daisy ▁ and ▁ Mr ▁. ▁ Rabbit ▁ had ▁ plenty ▁ of ▁ fun ▁ exploring ▁ the ▁ garden ▁ together ▁, ▁ until ▁ the ▁ sun ▁ set ▁ and ▁ it ▁ was ▁ time █ for ▁ Daisy ▁ to ▁ go ▁ home ▁. ▁
Click to see all of example 8
Once ▁ upon ▁ a ▁ time ▁ there ▁ was ▁ a ▁ quiet ▁ sheep ▁ called ▁ Daisy ▁, ▁ who ▁ liked ▁ to ▁ wander ▁ about ▁ in ▁ the ▁ grass ▁y ▁ fields ▁ by ▁ her ▁ farm ▁ house ▁. ▁ One ▁ day ▁, ▁ Daisy ▁ boldly ▁ followed ▁ the ▁ sun ▁ as ▁ it ▁ started ▁ to ▁ set ▁ and ▁ she ▁ ended ▁ up ▁ at ▁ a ▁ tall ▁, ▁ leather ▁ gate ▁. ▁ ▁\n ▁\n ▁The ▁ gate ▁ had ▁ an ▁ old ▁, ▁ heavy ▁ pad ▁lock ▁ on ▁ it ▁ that ▁ Daisy ▁ could ▁ not ▁ open ▁. ▁ Suddenly ▁, ▁ she ▁ heard ▁ a ▁ friendly ▁ voice ▁ coming ▁ up ▁ from ▁ behind ▁ her ▁, ▁ � ▁� ▁€ ▁� ▁� ▁Would ▁ you ▁ like ▁ me ▁ to ▁ show ▁ you ▁ what ▁ is ▁ behind ▁ this ▁ gate ▁? ▁â ▁€ ▁\n ▁\n ▁Da ▁isy ▁ was ▁ startled ▁ and ▁ turned ▁ around ▁ to ▁ see ▁ a ▁ white ▁ rabbit ▁ with ▁ long ▁ ears ▁ and ▁ a ▁ long ▁, ▁ leather ▁ tail ▁. ▁ Daisy ▁ was ▁ happy ▁ to ▁ meet ▁ the ▁ rabbit ▁, ▁ who ▁ introduced ▁ himself ▁ as ▁ Mr ▁. ▁ Rabbit ▁. ▁\n ▁\n ▁Mr ▁. ▁ Rabbit ▁ explained ▁, ▁ � ▁� ▁€ ▁� ▁� ▁If ▁ you ▁ follow ▁ me ▁, ▁ I ▁ will ▁ lead ▁ you ▁ to ▁ a ▁ special ▁ place ▁ through ▁ the ▁ gate ▁ and ▁ you ▁ can ▁ discover ▁ what ▁ is ▁ around ▁ the ▁ bend ▁â ▁€ ▁. ▁ ▁\n ▁\n ▁Da ▁isy ▁ followed ▁ the ▁ rabbit ▁ beyond ▁ the ▁ leather ▁ gate ▁, ▁ not ▁ knowing ▁ what ▁ was ▁ to ▁ come ▁. ▁ The ▁ path ▁ was ▁ quiet ▁ as ▁ the ▁ two ▁ friends ▁ walked ▁ along ▁, ▁ until ▁ they ▁ came ▁ upon ▁ a ▁ wond ▁rous ▁ field ▁. ▁ ▁\n ▁\n ▁Del ▁ight ▁ed ▁, ▁ Daisy ▁ shouted ▁ for ▁ joy ▁, ▁ for ▁ she ▁ had ▁ discovered ▁ the ▁ most ▁ beautiful ▁ garden ▁ she ▁ had ▁ ever ▁ seen ▁. ▁ Daisy ▁ and ▁ Mr ▁. ▁ Rabbit ▁ had ▁ plenty ▁ of ▁ fun ▁ exploring ▁ the ▁ garden ▁ together ▁, ▁ until ▁ the ▁ sun ▁ set ▁ and ▁ it ▁ was ▁ time █ for ▁ Daisy ▁ to ▁ go ▁ home ▁. ▁ ▁\n ▁\n ▁Da ▁isy ▁ thanked ▁ Mr ▁. ▁ Rabbit ▁ for ▁ leading ▁ her ▁ to ▁ this ▁ special ▁ place ▁ and ▁ waved ▁ goodbye ▁ to ▁ him ▁ as ▁ she ▁ hopped ▁ away ▁, ▁ content ▁
Example 9: She ▁ ran ▁ around ▁ and ▁ had ▁ lots ▁ of ▁ fun ▁. ▁ When ▁ it ▁ was ▁ time █ to ▁ go ▁ back ▁ home ▁, ▁
Click to see all of example 9
Once ▁ there ▁ was ▁ a ▁ brave ▁ child ▁ named ▁ Tina ▁. ▁ She ▁ wanted ▁ to ▁ go ▁ on ▁ an ▁ adventure ▁ to ▁ visit ▁ a ▁ special ▁ square ▁. ▁ So ▁ she ▁ put ▁ on ▁ her ▁ red ▁ coat ▁ and ▁ went ▁ out ▁ the ▁ door ▁. ▁ ▁\n ▁\n ▁On ▁ the ▁ way ▁, ▁ she ▁ met ▁ a ▁ friendly ▁ bird ▁. ▁ The ▁ bird ▁ ch ▁ir ▁ped ▁, ▁ � ▁� ▁€ ▁� ▁� ▁Where ▁ are ▁ you ▁ going ▁? ▁â ▁€ ▁ ▁\n ▁\n ▁â ▁€ ▁� ▁� ▁I ▁ am ▁ going ▁ to ▁ visit ▁ a ▁ special ▁ square ▁, ▁â ▁€ ▁ said ▁ Tina ▁ brave ▁ly ▁. ▁ ▁\n ▁\n ▁The ▁ bird ▁ said ▁, ▁ � ▁� ▁€ ▁� ▁� ▁I ▁ can ▁ show ▁ you ▁ the ▁ way ▁. ▁ Follow ▁ me ▁! ▁â ▁€ ▁ So ▁ Tina ▁ followed ▁ the ▁ bird ▁ to ▁ the ▁ square ▁. ▁ ▁\n ▁\n ▁When ▁ she ▁ arrived ▁, ▁ Tina ▁ saw ▁ a ▁ big ▁ park ▁ and ▁ a ▁ playground ▁. ▁ Her ▁ eyes ▁ lit ▁ up ▁ with ▁ joy ▁. ▁ She ▁ ran ▁ around ▁ and ▁ had ▁ lots ▁ of ▁ fun ▁. ▁ When ▁ it ▁ was ▁ time █ to ▁ go ▁ back ▁ home ▁, ▁ Tina ▁ thanked ▁ the ▁ bird ▁ for ▁ showing ▁ her ▁ the ▁ way ▁. ▁ ▁\n ▁\n ▁The ▁ bird ▁ waved ▁ goodbye ▁ and ▁ said ▁, ▁ � ▁� ▁€ ▁� ▁� ▁Good ▁bye ▁, ▁ brave ▁ Tina ▁. ▁ I ▁ hope ▁ you ▁ will ▁ visit ▁ us ▁ again ▁! ▁â ▁€ ▁\n ▁\n ▁T ▁ina ▁ smiled ▁ and ▁ said ▁, ▁ � ▁� ▁€ ▁� ▁� ▁Yes ▁, ▁ I ▁ will ▁! ▁â ▁€ ▁ Then ▁ she ▁ waved ▁ goodbye ▁ and ▁ went ▁ back ▁ home ▁. ▁
Example 10: It ▁ was ▁ so ▁ much ▁ fun ▁! ▁ They ▁ even ▁ saw ▁ some ▁ ducks ▁ swimming ▁ around ▁ them ▁. ▁\n ▁\n ▁After ▁ a ▁ while ▁, ▁ the ▁ brothers ▁ decided ▁ it ▁ was ▁ time █ to ▁ go ▁ back ▁ home ▁. ▁
Click to see all of example 10
Once ▁ upon ▁ a ▁ time ▁ there ▁ were ▁ two ▁ brothers ▁, ▁ Harry ▁ and ▁ Rory ▁. ▁ One ▁ day ▁, ▁ the ▁ two ▁ brothers ▁ were ▁ walking ▁ by ▁ the ▁ lake ▁ when ▁ they ▁ saw ▁ something ▁ floating ▁ on ▁ the ▁ lake ▁ - ▁ it ▁ was ▁ a ▁ raft ▁. ▁\n ▁\n ▁Harry ▁ asked ▁ Rory ▁, ▁ “ ▁What ▁ is ▁ that ▁?“ ▁\n ▁\n ▁R ▁ory ▁ replied ▁, ▁ “ ▁I ▁ think ▁ it ▁ is ▁ a ▁ raft ▁.“ ▁\n ▁\n ▁Harry ▁ was ▁ excited ▁ and ▁ said ▁, ▁ “ ▁Let ▁’s ▁ go ▁ see ▁!“ ▁\n ▁\n ▁So ▁ the ▁ two ▁ brothers ▁ went ▁ over ▁ to ▁ the ▁ raft ▁. ▁ When ▁ they ▁ got ▁ close ▁, ▁ they ▁ saw ▁ a ▁ big ▁ smile ▁y ▁ face ▁ painted ▁ on ▁ the ▁ raft ▁. ▁ They ▁ knew ▁ they ▁ were ▁ lucky ▁! ▁\n ▁\n ▁The ▁ two ▁ brothers ▁ climbed ▁ onto ▁ the ▁ raft ▁ and ▁ began ▁ to ▁ paddle ▁ around ▁ the ▁ lake ▁. ▁ It ▁ was ▁ so ▁ much ▁ fun ▁! ▁ They ▁ even ▁ saw ▁ some ▁ ducks ▁ swimming ▁ around ▁ them ▁. ▁\n ▁\n ▁After ▁ a ▁ while ▁, ▁ the ▁ brothers ▁ decided ▁ it ▁ was ▁ time █ to ▁ go ▁ back ▁ home ▁. ▁ But ▁ before ▁ they ▁ could ▁ get ▁ off ▁ the ▁ raft ▁, ▁ Harry ▁ said ▁, ▁ “ ▁Wait ▁, ▁ I ▁ forgot ▁ something ▁!“ ▁\n ▁\n ▁R ▁ory ▁ asked ▁, ▁ “ ▁What ▁ did ▁ you ▁ forget ▁?“ ▁\n ▁\n ▁Harry ▁ said ▁, ▁ “ ▁I ▁ found ▁ something ▁ on ▁ the ▁ raft ▁!“ ▁\n ▁\n ▁R ▁ory ▁ looked ▁ around ▁ and ▁ could ▁ not ▁ find ▁ anything ▁. ▁ But ▁ then ▁ Harry ▁ pulled ▁ out ▁ a ▁ big ▁ red ▁ ball ▁ from ▁ under ▁ the ▁ edge ▁ of ▁ the ▁ raft ▁. ▁ It ▁ was ▁ a ▁ lucky ▁ find ▁! ▁\n ▁\n ▁The ▁ two ▁ brothers ▁ waved ▁ goodbye ▁ to ▁ the ▁ raft ▁ and ▁ took ▁ the ▁ ball ▁ home ▁. ▁ They ▁ had ▁ a ▁ lot ▁ of ▁ fun ▁ and ▁ they ▁ were ▁ so ▁ lucky ▁ to ▁ find ▁ the ▁ ball ▁! ▁
Dead features
On 1000 examples from the validation set, each of the autoencoder’s 10000 features activated at least once, i.e. there were no dead features.
My understanding of the literature is that dead features are more of a problem for larger autoencoders:
At the end of training, we defined “dead” features as those which were not active over a sample of \(10^{7}\) tokens. The proportion of dead features was roughly 2% for the 1M SAE, 35% for the 4M SAE, and 65% for the 34M SAE.
Automatic feature examination
Manually judging if features are interpretable doesn’t scale. So I’ve outsourced the work to GPT-4o, with the following prompts:
System prompt:
You are a helpful assistant.
User prompt:
I’ll send you a sequence of texts, where tokens have been highlighted using Unicode Block Elements. Look for a pattern in which tokens get strong highlights. Rank how clear the pattern is on a 1-5 scale, where 1 is no detectable pattern, 3 is a vague pattern with some exceptions, and 5 is a clear pattern with no exceptions. Focus on the pattern in the strong highlights. Describe the pattern in 10 words or less“
Let’s also ask GPT-4o to rank how interpretable the original language model activations are (same prompt). Hence we can compare to the sparse autoencoder’s features, which ideally GPT-4o will rate as much more clear.
Finicky detail: The LM activations can be positive or negative, unlike the always nonnegative autoencoder features. We want the activations to be nonnegative, so that 0 will be “weakly activating” and a large positive number will be “strongly activating”.
Three ways we could do this:
- Take the ReLU, i.e. set negative activations to 0
- Take the absolute value
- Use an affine shift, i.e. add the smallest possible number to all the acitvations so that the least activation is now 0
I don’t think there’s a strong justification for choosing one of these methods over another, so I’ve implemented them all.
Results:
Discussion
The results are directionally as expected: GPT-4o ranks the autoencoder features as clearer: less likely to be 3/5 than the LLM activations, more likely to be 5/5.
It would be nice to have a reliable automated interpretability system, which would allow extensions like “ if we trained for half as long, is the autoencoder half as interpretable?“ But my inclination is to not fully trust my current automated system because of the caveats discussed below.
Caveat 1
What I call “language model activations” are the residual stream activations, i.e. the exact tensors I trained the SAE on Anthropic instead compared their autoencoder’s interpretability to neurons in Claude’s previous MLP layer.
There’s an argument that residual stream is less likely to have interpretable activations as-is, since the addition mixes the outputs of earlier neurons together. (“As-is” means that no autoencoder/mechanistic interpretability tools are required.)
My prior is that the MLP neurons for roneneldan/TinyStories-33M
wouldn’t be interpretable either,
but that’s definitely worth looking into.
Caveat 2
My anecdotal impression from looking through the results is that GPT-4o is a lenient grader. For instance, GPT-4o gave a clearness score of 4/5 to the LM’s 0th residual activation (the affine case), and it found the pattern “Strong highlights emphasize dialogue and reactions.” I disagree with this score, since a typical example of this LM activation has most tokens strongly highlighted, unlike the autoencoder’s sparsity. For example:
Once ▄ upon ▃ a ▃ time ▄, ▄ there ▄ was ▄ a ▄ huge ▃ bear ▃ called ▄ Peter ▄. ▄ Peter ▄ lived ▃ deep ▂ in ▄ the ▄ forest ▅ in ▄ a ▄ big ▃, ▄ cos ▄y ▂ cave ▄. ▄ ▄ ▄One ▄ day ▅, ▄ Peter ▄ was ▄ feeling ▃ tired ▅ and ▄ decided ▄ he ▄ should ▃ go ▅ to ▆ sleep ▆. ▄ So ▅ he ▅ climbed ▄ into ▆ his ▅ bed ▆ and ▅ closed ▄ his ▄ eyes ▆. ▄ Suddenly ▅, ▅ he ▅ heard ▆ a ▆ noise ▆ outside █ his ▄ cave ▅. ▄ “ ▅What ▅ was ▆ that ▄?“ ▄ he ▅ thought ▄. ▅ ▅ ▅Peter ▄ went ▄ outside ▆ to ▅ investigate ▅. ▅ He ▆ saw ▅ a ▄ little ▄ mouse ▃, ▄ and ▄ the ▅ mouse ▄ was ▄ in ▃ trouble ▅! ▄ Its ▄ tail ▄ was ▄ stuck ▃ under ▄ a ▃ big ▄ rock ▄ and ▄ it ▄ couldn ▄’t ▅ get ▄ free ▄. ▄ ▅ ▄“ ▅Don ▄’t ▃ worry ▃,“ ▃ said ▅ Peter ▄. ▄ “ ▅I ▄’ll ▂ help ▅ you ▃!“ ▄ He ▅ used ▅ all ▄ his ▅ strength ▃ to ▄ move ▅ the ▄ huge ▄ rock ▄ and ▅ the ▅ mouse ▃ ran ▄ away ▄. ▄ ▅ ▄Peter ▄ smiled ▃, ▄ but ▄ he ▅ was ▄ very ▄ tired ▄ now ▄. ▄ He ▅ said ▅ goodbye ▅ to ▆ the ▅ mouse ▂ and ▄ went ▄ back ▅ to ▅ his ▅ cave ▅. ▄ He ▅ was ▄ so ▄ exhausted ▄, ▃ he ▄ fell ▃ asleep ▅ right ▄ away ▄. ▄ ▅ ▄From ▄ that ▃ day ▄ on ▄, ▄ Peter ▄ and ▅ the ▅ mouse ▃ were ▄ good ▃ friends ▃. ▄ They ▅ always ▄ looked ▄ out ▇ for ▄ each ▃ other ▃ and ▄ helped ▄ each ▂ other ▄ if ▁ they ▅ got ▄ into ▅ trouble ▄. ▄ And ▄ they ▅ both ▄ slept ▄ well ▄ each ▃ night ▅, ▄ knowing ▄ they ▄ had ▄ a ▄ friend ▄ who ▅ would ▃ help ▄ them ▄ out ▄ if ▂ they ▅ needed ▅ it ▄! ▄
The strongest activating token in the above example is “outside” in “he heard a noise outside”, which is neither a dialogue or a reaction.
What could have gone wrong here?
- Maybe more prompt engineering is needed. I could tell GPT-4o “be strict” or “if most tokens have strong highlights, rate it 1/5”.
- Maybe reading Unicode block elements is trickier for an LLM, and a different format (e.g. keep the activation strength as a floating point number) might be easier for it to comprehend.
- Or maybe the prompt should be split in two:
- First ask GPT-4o to describe a pattern given the examples
- Then ask it (with a blank context) to decide which of two examples (a real one and a distractor) exhibits the pattern. Ideally this would detect when GPT-4o has wrongly found a generic pattern that could match any example
Steering
We want to also see a causal effect: If an autoencoder feature is on when there’s a certain pattern in the text, can we make that pattern appear in generated text by turning the feature on?
Specifically, for a feature i, we set
nudge
to be the ith vector in the autoencoder’s decoder_linear
weight matrix.
Recall that this weight matrix is a tensor with dimensions (10000, 768)
(number of autoencoder features, dimension of the LM’s residual activations).
So nudge
is in
\(\mathbb{R}^{768} \).
Then we use the LM to generate text,
except at each autoregressive step we
add 10 * nudge
to the residual activation
after the 2nd layer (i.e. the same layer the autoencoder was trained on).
Anecdotally I’ve found that
10 * nudge
makes steering more likely to work than nudge
,
although at the cost of some text quality. We prompt the LM with “Once upon a time”.
(The above algorithm is similar to, but not the same as, the clamping Anthropic describes here.)
To get the ith feature vector,
It’s tempting to instead define
nudge = sae.decoder(onehot)
,
where onehot = torch.tensor([0,...,0,1,0,...,0])
is a unit vector with a 1
in the ith place.
The problem is that sae.decoder
multiplies by the weight matrix
(picking out the correct feature vector) but also adds the bias.
This bias is large enough to mess up steering, i.e.
adding in this corrupted nudge
doesn’t make the model generate
text that resembles the desired feature.
After the initial tuning to get the scale factor 10, I generated two steered examples (limited to 100 tokens) for each of features 0, 1, 2, and 6, without cherrypicking the generations. Note that I’ve specifically chosen feature 6 since it’s an interesting case.
After generating the text, I fed it back into the unsteered LM, and used the autoencoder to check whether the feature activated, using the same block element format as here. This is a sanity check: If steering pushes the generated text towards exhibiting feature i’s pattern, the text should make feature i activate. These highlighted texts are hidden by default, but you can click to see them.
Feature 0
I said, “This feature activates on the quotation mark when someone starts talking”. Steering is a complete failure here. If you look at the highlights, feature 0 doesn’t even activate on the one quotation mark in the second generation.
Once upon a time, there was a little boy named Tim. He went on vacation with his mom, who put on a big bed. in small jail, so she said thank you. Tim was in jail, but in her while she was. They had to get in their room and make a big, clear room. Tim was so happy to enter the room and make everything in. After the door,, Tim was in a room with many passages. She,thanks for her home
Click to see the version with feature activations highlighted
Once ▁ upon ▁ a ▁ time ▁, ▁ there ▁ was ▁ a ▁ little ▁ boy ▁ named ▁ Tim ▁. ▁ He ▁ went ▁ on ▁ vacation ▁ with ▁ his ▁ mom ▁, ▁ who ▁ put ▁ on ▁ a ▁ big ▁ bed ▁. ▁ in ▁ small ▁ jail ▁, ▁ so ▁ she ▁ said ▁ thank ▁ you ▁. ▁ Tim ▁ was ▁ in ▁ jail ▁, ▁ but ▁ in ▁ her ▁ while ▁ she ▁ was ▁. ▁ They ▁ had ▁ to ▁ get ▁ in ▁ their ▁ room ▁ and ▁ make ▁ a ▁ big ▁, ▁ clear ▁ room ▁. ▁ Tim ▁ was ▁ so ▁ happy ▁ to ▁ enter █ the ▁ room ▁ and ▁ make ▁ everything ▁ in ▁. ▁ ▁ ▁After ▁ the ▁ door ▁, ▁, ▁ Tim ▁ was ▁ in ▁ a ▁ room ▁ with ▁ many ▁ passages ▁. ▁ She ▁, ▁thanks ▁ for ▁ her ▁ home ▁
Once upon a time, there was a little girl named Lily. She had a silver crown and her brother had won before.. She was a good boy, who wincedog means so smooth in.“ Lily and her brother were both so rich. They had, and the big brother made her smile. Even though she had to leave, like in jail, rich to get in with Ben. They both had surprise, and had to say goodbye, so they got
Click to see the version with feature activations highlighted
Once ▁ upon ▁ a ▁ time ▁, ▁ there ▁ was ▁ a ▁ little ▁ girl ▁ named ▁ Lily ▁. ▁ She ▁ had ▁ a ▁ silver ▁ crown ▁ and ▁ her ▁ brother ▁ had ▁ won ▁ before ▁. ▁. ▁ She ▁ was ▁ a ▁ good ▁ boy ▁, ▁ who ▁ win █ced ▁og ▁ means ▁ so ▁ smooth ▁ in ▁.“ ▁ ▁ ▁ ▁L ▁ily ▁ and ▁ her ▁ brother ▁ were ▁ both ▁ so ▁ rich ▁. ▁ They ▁ had ▁, ▁ and ▁ the ▁ big ▁ brother ▁ made ▁ her ▁ smile ▁. ▁ Even ▁ though ▁ she ▁ had ▁ to ▁ leave ▁, ▁ like ▁ in ▁ jail ▁, ▁ rich ▁ to ▁ get ▁ in ▁ with ▁ Ben ▁. ▁ ▁ ▁ ▁They ▁ both ▁ had ▁ surprise ▁, ▁ and ▁ had ▁ to ▁ say ▁ goodbye ▁, ▁ so ▁ they ▁ got ▁
Feature 1
I said, “This feature activates on a list of concrete nouns, almost always in the context of characters wondering about this list or playing pretend with the list.” Steering is somewhat successful here: there are lists like “a cloud, a small bird” or “a game or a pretend game”. And feature 1 then activates on those lists.
Once upon a time, there was a little bird, and each day. One day, when it was a bit of a wind, when it saw a cloud, a small bird. Then, a lot of more. Then, it learned something new. Every day and every morning, and every night, until it had two weeks of. many months and months. Finally, when it stopped, but mom and dad. Then, many more.
Click to see the version with feature activations highlighted
Once ▁ upon ▁ a ▁ time ▁, ▁ there ▁ was ▁ a ▁ little ▁ bird ▁, ▁ and ▁ each ▁ day ▁. ▁ ▁ ▁One ▁ day ▁, ▁ when ▁ it ▁ was ▁ a ▁ bit ▁ of ▁ a ▁ wind ▁, ▁ when ▁ it ▁ saw ▁ a ▁ cloud ▁, ▂ a ▅ small ▆ bird ▁. ▁ ▁ ▁Then ▁, ▁ a ▁ lot ▁ of ▁ more ▁. ▁ Then ▁, ▁ it ▁ learned ▁ something ▁ new ▁. ▁ ▁ ▁Every ▁ day ▁ and ▂ every ▅ morning ▁, ▁ and ▁ every █ night ▁, ▂ until ▁ it ▁ had ▁ two ▁ weeks ▁ of ▁. ▁ ▁ many ▁ months ▁ and ▁ months ▁. ▁ ▁ ▁Finally ▁, ▁ when ▁ it ▁ stopped ▁, ▁ but ▁ mom ▁ and ▁ dad ▁. ▁ ▁ ▁Then ▁, ▁ many ▁ more ▁. ▁
Once upon a time, there was a wise old man who liked to travel. He liked to eat, he was very curious and he wanted a new, sweet sometimes. He loved to learn new things. Every time he visited a new place, he wanted to watch television, or guess some more. He sometimes played a game or a pretend game. Then he guessed a story, and made some more games. Eventually, it was very fun. “Are so many
Click to see the version with feature activations highlighted
Once ▁ upon ▁ a ▁ time ▁, ▁ there ▁ was ▁ a ▁ wise ▁ old ▁ man ▁ who ▁ liked ▁ to ▁ travel ▁. ▁ He ▁ liked ▁ to ▁ eat ▁, ▁ he ▁ was ▁ very ▁ curious ▁ and ▁ he ▁ wanted ▁ a ▁ new ▁, ▁ sweet ▁ sometimes ▁. ▁ He ▁ loved ▁ to ▁ learn ▁ new ▁ things ▁. ▁ ▁ ▁ ▁Every ▁ time ▁ he ▁ visited ▁ a ▁ new ▁ place ▁, ▁ he ▁ wanted ▁ to ▁ watch ▁ television ▁, ▁ or ▁ guess ▁ some ▁ more ▁. ▁ He ▁ sometimes ▁ played ▁ a ▁ game ▁ or ▂ a █ pretend ▂ game ▄. ▁ Then ▁ he ▁ guessed ▁ a ▁ story ▁, ▂ and ▁ made ▁ some ▁ more ▁ games ▁. ▁ ▁ ▁Eventually ▁, ▁ it ▁ was ▁ very ▁ fun ▁. ▁ ▁ ▁“ ▁Are ▁ so ▁ many ▁
Feature 2
I said, “This feature activates on ‘time’ in ‘it was time to/for’, usually in a phrase like ‘the characters were having fun, but then it was time for something less fun’.”
In the first generation, the model does generate “time to”, although not after “it was”. So that’s a partial success. The second generation is another complete failure, and feature 2 doesn’t activate anywhere on it.
Once upon a time, there was a group to play with to a group for a long time to for the one to go to to the nation for a parade. “Time to go for the parade to for the parade to for the last to go for the Wheel. to the Wheel to the room for the Wheel. to remove the group for the tank to to exit for the to to exit. As for the for the group to go, the excited for to be for the new exit
Click to see the version with feature activations highlighted
Once ▁ upon ▁ a ▁ time ▁, ▁ there ▁ was ▁ a ▁ group ▁ to ▁ play ▁ with ▁ to ▁ a ▁ group ▁ for ▁ a ▁ long ▁ time ▁ to ▁ for ▁ the ▁ one ▁ to ▁ go ▁ to ▁ to ▁ the ▁ nation ▁ for ▁ a ▁ parade ▁. ▁ “ ▁Time █ to ▁ go ▁ for ▁ the ▁ parade ▁ to ▁ for ▁ the ▁ parade ▁ to ▁ for ▁ the ▁ last ▁ to ▁ go ▁ for ▁ the ▁ Wheel ▁. ▁ to ▁ the ▁ Wheel ▁ to ▁ the ▁ room ▁ for ▁ the ▁ Wheel ▁. ▁ to ▁ remove ▁ the ▁ group ▁ for ▁ the ▁ tank ▁ to ▁ to ▁ exit ▁ for ▁ the ▁ to ▁ to ▁ exit ▁. ▁ ▁ ▁As ▁ for ▁ the ▁ for ▁ the ▁ group ▁ to ▁ go ▁, ▁ the ▁ excited ▁ for ▁ to ▁ be ▁ for ▁ the ▁ new ▁ exit ▁
Once upon a time, there was a family for dinner. They all sat down for dinner for the meal to eat. Before dinner for dinner, for dinner to be for dinner for everyone to eat. Mom to order the family to eat to eat dinner for dinner. After dinner, for a full meal for dinner for the family to eat for dinner. for dinner for dessert their meal for them to be for dinner every year where they were for the dessert. The day to
Click to see the version with feature activations highlighted
Once ▁ upon ▁ a ▁ time ▁, ▁ there ▁ was ▁ a ▁ family ▁ for ▁ dinner ▁. ▁ They ▁ all ▁ sat ▁ down ▁ for ▁ dinner ▁ for ▁ the ▁ meal ▁ to ▁ eat ▁. ▁ ▁ ▁Before ▁ dinner ▁ for ▁ dinner ▁, ▁ for ▁ dinner ▁ to ▁ be ▁ for ▁ dinner ▁ for ▁ everyone ▁ to ▁ eat ▁. ▁ Mom ▁ to ▁ order ▁ the ▁ family ▁ to ▁ eat ▁ to ▁ eat ▁ dinner ▁ for ▁ dinner ▁. ▁ ▁ ▁After ▁ dinner ▁, ▁ for ▁ a ▁ full ▁ meal ▁ for ▁ dinner ▁ for ▁ the ▁ family ▁ to ▁ eat ▁ for ▁ dinner ▁. ▁ for ▁ dinner ▁ for ▁ dessert ▁ their ▁ meal ▁ for ▁ them ▁ to ▁ be ▁ for ▁ dinner ▁ every ▁ year ▁ where ▁ they ▁ were ▁ for ▁ the ▁ dessert ▁. ▁ ▁ ▁The ▁ day ▁ to ▁
I looked at more generations with feature 2, and “dinner” appears often. I’m uncertain if this is coincidence or if there is an explanation (whereas for feature 6 below, I found an explanation).
Feature 6
In the top 10 examples (ranked by strength of activation) from the TinyStories validation set, feature 6 only activated on “fire” (or more weakly on “firemen”). And yet these generations aren’t related to fire at all:
Once upon a time, there was an ignorant driver. One day, the cars that was traveling quickly, and so did a terrible crash. The lights went so quick ly, that was very loud. <|endoftext|>
Click to see the version with feature activations highlighted
Once ▁ upon ▁ a ▁ time ▁, ▁ there ▁ was ▁ an ▁ ignorant ▁ driver ▁. ▁ One ▁ day ▁, ▁ the ▁ cars █ that ▁ was ▁ traveling ▁ quickly ▁, ▁ and ▁ so ▁ did ▁ a ▁ terrible ▁ crash ▇. ▁ The ▁ lights ▁ went ▅ so ▁ quickly ▁, ▁ that ▁ was ▁ very ▁ loud ▂. ▁ ▁<|endoftext|> ▁
Once upon a time, there was a train. On one of the roads, a very big crash happened. It made a lot of noise. “”. crash the cars and truck.,“ and cars,“ crash with a loud noise. That noise and accident. One day, red and noisy cars came to distant. The river, which is really a rough and dangerous. Jark. The people in the traffic and noise and traffic crash, ever. The red
Click to see the version with feature activations highlighted
Once ▁ upon ▁ a ▁ time ▁, ▁ there ▁ was ▁ a ▁ train ▂. ▁ On ▁ one ▁ of ▁ the ▁ roads ▁, ▁ a ▁ very ▁ big ▁ crash ▆ happened ▁. ▁ It ▁ made ▁ a ▁ lot ▁ of ▁ noise ▂. ▁ “ ▁“. ▁ crash ▆ the ▁ cars ▄ and ▁ truck ▁. ▁,” ▁ and ▁ cars ▃,“ ▁ crash ▄ with ▁ a ▁ loud ▁ noise ▂. ▁ That ▁ noise ▅ and ▁ accident ▃. ▁ ▁ ▁One ▁ day ▁, ▁ red ▁ and ▁ noisy ▂ cars ▃ came ▁ to ▁ distant ▁. ▁ The ▁ river ▅, ▁ which ▂ is ▁ really ▁ a ▁ rough ▁ and ▁ dangerous ▂. ▁ ▁J ▂ark ▁. ▁ ▁ ▁The ▁ people ▁ in ▁ the ▁ traffic ▅ and ▁ noise ▄ and ▁ traffic ▆ crash █, ▁ ever ▁. ▁ ▁ ▁ ▁The ▁ red ▁
If you look at the highlights, you’ll see that feature 6 is activating, but on unrelated words like “crash” and “cars”. A natural hypothesis is that feature 6 isn’t a “fire” feature, but instead a “dangerous thing” feature.
If we look at examples from the validation set that don’t activate quite as strongly as the top 10, we indeed see that feature 6 activates on “earthquake” and “flood”:
Excerpt 1:
“ ▁Mom ▁my ▁, ▁ what ▁’s ▁ happening ▁?“ ▁ she ▁ cried ▁. ▁ “ ▁It ▁’s ▁ an ▁ earthquake ▆, ▁ Lily ▁,“ ▁ her ▁ mom ▁my ▁ said ▁
Excerpt 2:
Tim ▁my ▁’s ▁ mom ▁my ▁ told ▁ him ▁ to ▁ come ▁ inside ▁ because ▁ there ▁ might ▁ be ▁ a ▁ flood ▇. ▁ Tim ▁my ▁ didn ▁’t ▁ know ▁ what ▁ a ▁ flood █ was ▁, ▁ but ▁ he ▁ listened ▁ to ▁ his ▁ mom ▁my ▁ and ▁ went ▁ inside ▁. ▁
I speculate that the LM thinks fire is the most dangerous of all dangerous things, so the top-activating examples were all fire.